Can Sample Variance Be Smaller Than Population Variance
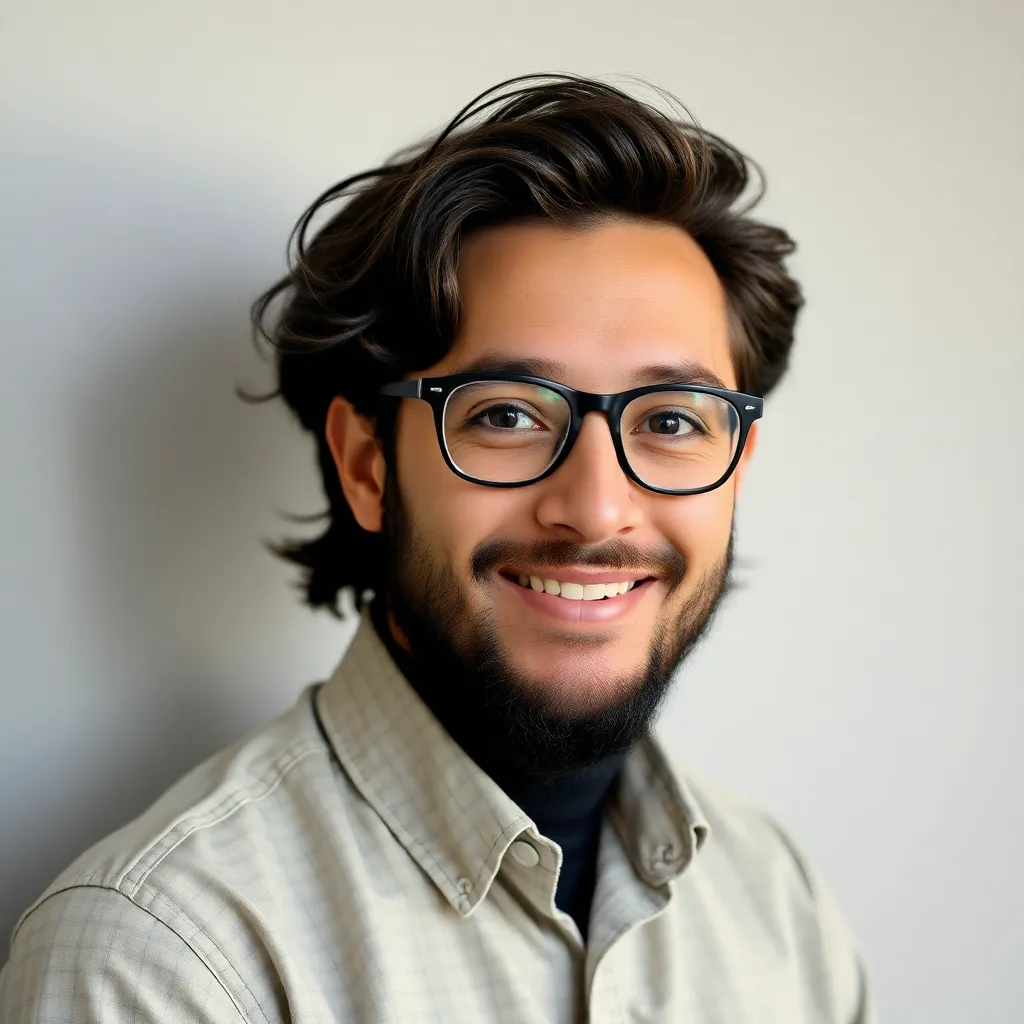
Arias News
Apr 15, 2025 · 6 min read

Table of Contents
Can Sample Variance Be Smaller Than Population Variance? A Deep Dive into Statistical Inference
Understanding the relationship between sample variance and population variance is crucial in statistics. While it's intuitive to assume that the sample variance will always underestimate the population variance, this isn't always true. This article delves into the intricacies of this relationship, exploring the conditions under which sample variance can indeed be smaller than population variance, and why this seemingly counterintuitive result arises.
Understanding Variance: Population vs. Sample
Before we explore the possibility of sample variance being smaller than population variance, let's establish a clear understanding of both concepts.
Population Variance: This represents the average squared deviation of each data point in the entire population from the population mean. It's a measure of the overall spread or dispersion within the population. We denote it as σ² (sigma squared). Calculating the population variance requires knowing every data point in the entire population, which is often impractical or impossible.
Sample Variance: This is an estimate of the population variance, calculated from a subset (sample) of the population data. It provides an approximation of the population's spread based on the available data. We denote it as s² (s squared). Since it's derived from a sample, it's subject to sampling error – the difference between the sample statistic and the true population parameter.
The formulas for calculating population and sample variances highlight a key difference:
-
Population Variance (σ²): Σ(xᵢ - μ)² / N, where xᵢ are individual data points, μ is the population mean, and N is the population size.
-
Sample Variance (s²): Σ(xᵢ - x̄)² / (n - 1), where xᵢ are individual data points, x̄ is the sample mean, and n is the sample size. Notice the denominator (n-1) instead of n. This is crucial for obtaining an unbiased estimator of the population variance.
The (n-1) Correction: Why it Matters
The use of (n-1) in the sample variance formula, known as Bessel's correction, is essential for obtaining an unbiased estimator of the population variance. Without this correction, the sample variance would systematically underestimate the population variance, especially for small sample sizes.
This correction accounts for the fact that a sample mean tends to be closer to the data points in the sample than the true population mean. Using 'n' in the denominator underestimates the variability because it doesn't fully account for the uncertainty introduced by estimating the population mean from the sample. By using (n-1), we inflate the sample variance slightly, making it a more accurate estimate of the population variance.
Can Sample Variance Be Smaller? Yes, but It's Less Likely
While Bessel's correction helps to mitigate underestimation, it doesn't guarantee that the sample variance will always be larger than the population variance. It is entirely possible, though less probable, for the sample variance to be smaller. This happens due to the inherent randomness of sampling. The sample you select might, by chance, contain data points that are clustered more tightly around the sample mean than the population data points are around the population mean. This random sampling effect creates the possibility for a lower sample variance.
Scenarios Where Sample Variance Might Be Smaller
Let's consider some specific scenarios to illustrate how sample variance could be smaller than population variance:
-
Unrepresentative Sample: If the sample is not a good representation of the population (e.g., it is biased or significantly skewed away from the population's true distribution), the sample variance might be unusually low. For instance, if you're sampling heights and accidentally select a disproportionate number of people of similar height, your sample variance will underestimate the population variance.
-
Sampling Error: Even with a representative sample, random chance can lead to a sample variance that is smaller than the population variance. This is more likely to occur with small sample sizes, where the impact of random fluctuations is magnified. Imagine drawing multiple samples from the same population; you'll observe variability in the calculated sample variance across the samples. Some samples will have lower variances than the population variance due to random sampling fluctuations.
-
Specific Distributions: The probability of observing a smaller sample variance depends on the underlying population distribution. For certain distributions (like those with heavy tails), there's a higher chance that a randomly drawn sample might have a lower variance compared to the population.
-
Outliers: If the population has substantial outliers, and the sample happens to exclude these outliers (purely by chance), the sample variance could be significantly smaller than the population variance. The presence of outliers significantly inflates the population variance.
Probability and Statistical Significance
The probability of obtaining a sample variance smaller than the population variance decreases as the sample size increases. With larger samples, the sample mean becomes a better estimate of the population mean, and the sample variance becomes a more reliable estimator of the population variance. The law of large numbers dictates that, as the sample size approaches the population size, the sample variance converges towards the population variance.
It's important to remember that even if the sample variance is smaller than the population variance, this doesn't necessarily imply that something is wrong with the sampling or analysis. It's simply a consequence of the inherent variability in sampling. The likelihood of this event occurring is small, but it's certainly within the realm of statistical possibility.
Importance of Proper Sampling Techniques
The possibility of obtaining a smaller sample variance highlights the critical importance of using appropriate sampling techniques. A biased or unrepresentative sample will lead to inaccurate estimates of the population parameters. Employing techniques like stratified sampling, cluster sampling, or random sampling helps to ensure that the sample accurately reflects the population and minimizes the chances of obtaining a grossly misleading sample variance.
Furthermore, a proper understanding of the limitations of sample statistics is crucial. While sample statistics provide valuable insights into population parameters, they are subject to sampling error. Researchers must interpret results cautiously, acknowledging the potential for random fluctuations and always reporting the sample size to allow readers to gauge the reliability of the findings.
Conclusion: Context Matters
While it's less common, a sample variance can indeed be smaller than the population variance. This isn't an indication of a flawed method but rather a reflection of the randomness inherent in sampling. However, the probability of this occurrence decreases as the sample size increases and when appropriate sampling techniques are employed. The key takeaway is that interpreting sample variance requires careful consideration of the sampling methodology, sample size, and the underlying population distribution. Always remember to contextualize your findings and avoid drawing oversimplified conclusions based solely on the relative magnitudes of sample and population variances. Understanding these nuances is critical for robust statistical inference and for drawing reliable conclusions from data analysis. The possibility of this seemingly counterintuitive result underscores the complexity and fascinating nature of statistical inference.
Latest Posts
Latest Posts
-
How Many Times Does 8 Go Into 56
Apr 16, 2025
-
How Much Does A Pressure Treated 6x6x12 Weight
Apr 16, 2025
-
How Do You Say Brandon In Spanish
Apr 16, 2025
-
How Old Was Jem In To Kill A Mockingbird
Apr 16, 2025
-
How Many Cubic Feet Is 50 Pounds Of Sand
Apr 16, 2025
Related Post
Thank you for visiting our website which covers about Can Sample Variance Be Smaller Than Population Variance . We hope the information provided has been useful to you. Feel free to contact us if you have any questions or need further assistance. See you next time and don't miss to bookmark.