Graphs Of The Data From Laboratory Investigations Are Used To
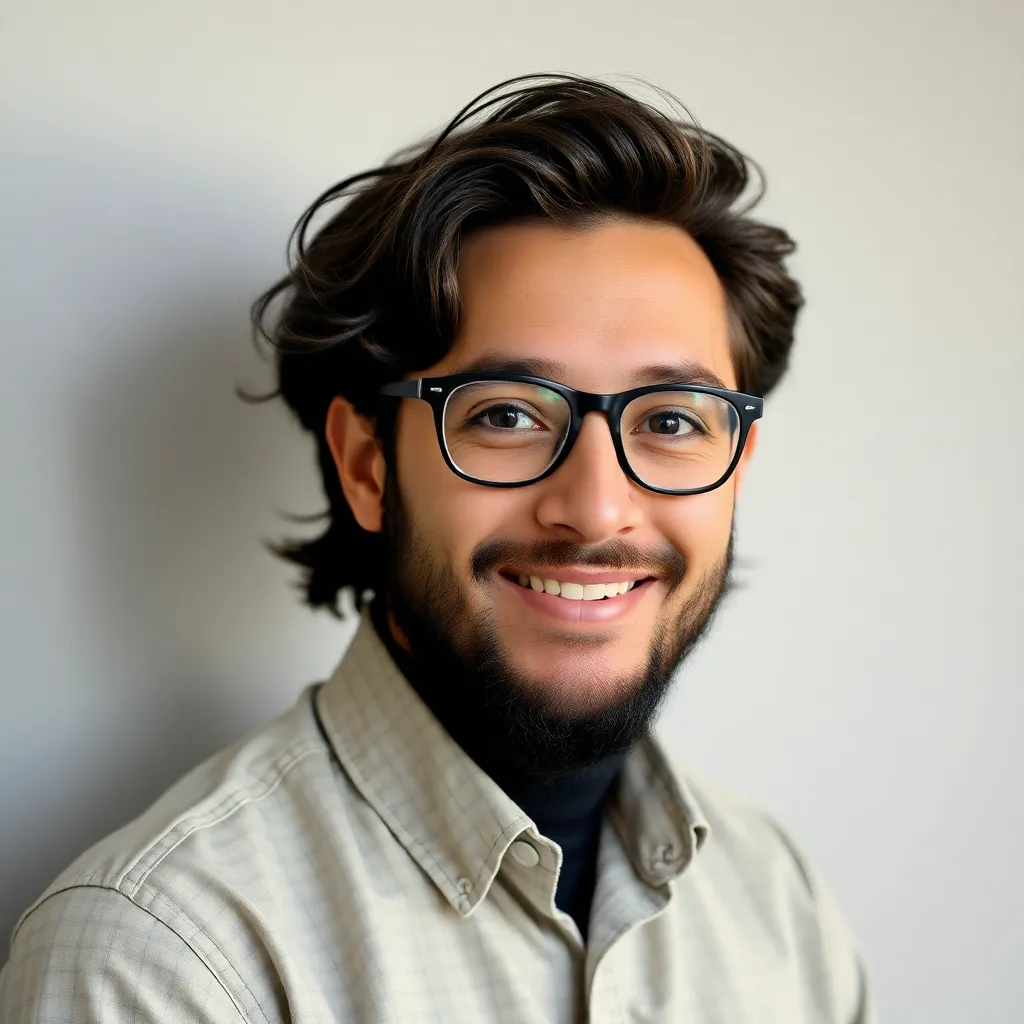
Arias News
May 10, 2025 · 7 min read
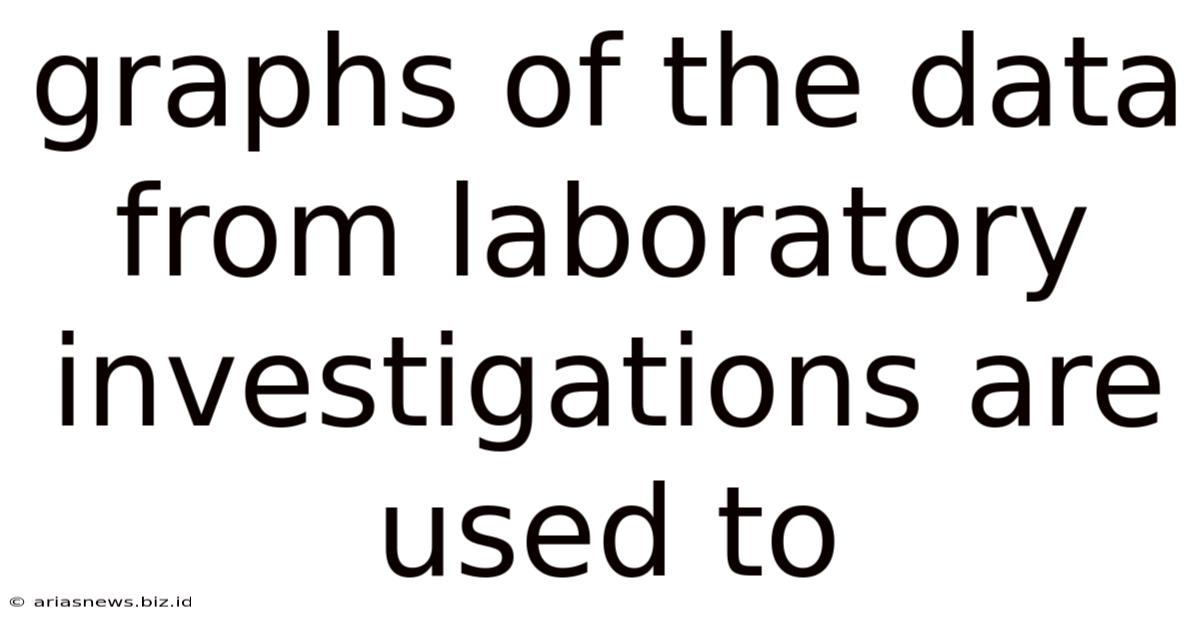
Table of Contents
Graphs of Data from Laboratory Investigations: Unveiling Insights and Trends
Graphs are indispensable tools in the realm of laboratory investigations. They transform raw data into visually compelling representations, revealing patterns, trends, and relationships that might otherwise remain hidden within spreadsheets of numbers. This comprehensive guide explores the myriad ways graphs are utilized to interpret, analyze, and communicate findings from diverse laboratory experiments and studies. We'll delve into the different types of graphs best suited for specific data types and discuss the crucial elements for creating clear, informative, and scientifically rigorous graphical representations.
Why Use Graphs in Laboratory Investigations?
The primary function of graphs in laboratory investigations is to visualize data. This visual representation offers several key advantages:
1. Enhanced Data Interpretation:
Numerical data alone can be overwhelming and difficult to interpret. Graphs provide a concise and easily digestible summary, allowing researchers to quickly identify trends, outliers, and significant relationships within the data. A quick glance at a well-constructed graph can reveal insights that would require extensive numerical analysis to uncover.
2. Improved Communication of Findings:
Graphs are exceptionally effective tools for communicating research findings to a broader audience. They transcend the limitations of purely textual descriptions, making complex data accessible to both experts and non-experts. A well-designed graph can effectively communicate key results and conclusions to colleagues, supervisors, reviewers, and even the general public.
3. Identification of Patterns and Trends:
Graphs facilitate the identification of patterns and trends within the data. Visual inspection of a graph can reveal linear relationships, exponential growth or decay, cyclical patterns, and other significant trends that might not be readily apparent from a table of numbers. These patterns are crucial for formulating hypotheses, testing theories, and drawing meaningful conclusions.
4. Detection of Anomalies and Outliers:
Graphs enable the easy detection of anomalies and outliers within a dataset. Outliers—data points that deviate significantly from the overall trend—can represent experimental errors, measurement inaccuracies, or genuinely unusual phenomena. Identifying these outliers is essential for assessing data quality and ensuring the reliability of the results.
5. Comparison of Different Datasets:
Graphs are highly effective for comparing different datasets. By plotting multiple datasets on the same graph, researchers can visually compare trends, magnitudes, and variations across different experimental groups, treatments, or time points. This visual comparison greatly aids in drawing meaningful conclusions about the relationships between variables.
6. Supporting Scientific Arguments:
Graphs are critical components of scientific reports, publications, and presentations. They provide visual evidence to support scientific arguments and conclusions. A graph can significantly strengthen a research paper or presentation by providing a clear and compelling visual representation of the data supporting the claims made.
Types of Graphs Commonly Used in Laboratory Investigations
The choice of graph type depends heavily on the nature of the data and the relationships being investigated. Here are some of the most commonly employed graph types in laboratory settings:
1. Line Graphs:
Line graphs are ideal for displaying continuous data showing changes over time or across a continuous variable. They are commonly used to represent kinetic data, growth curves, titration curves, and other data sets where the independent variable is continuous. Multiple lines can be plotted on the same graph to compare different treatments or conditions.
Example: Plotting the absorbance of a reaction mixture over time to study reaction kinetics.
2. Scatter Plots:
Scatter plots are used to illustrate the relationship between two variables. Each data point represents a single observation, and the pattern of points can reveal correlations, clusters, or other relationships between the variables. Scatter plots are often used to assess the correlation coefficient between two variables.
Example: Plotting enzyme activity against substrate concentration to determine the Michaelis-Menten constant.
3. Bar Graphs:
Bar graphs are best suited for representing categorical data or comparing the means of different groups. They are frequently used to compare the average values of a dependent variable across different experimental groups, treatments, or categories.
Example: Comparing the average growth rates of plants under different light conditions.
4. Histograms:
Histograms are used to show the distribution of a single continuous variable. They divide the data into intervals (bins) and display the frequency of data points within each interval. Histograms provide insights into the shape of the data distribution, such as its symmetry, skewness, and modality.
Example: Showing the distribution of particle sizes in a sample.
5. Pie Charts:
Pie charts are useful for displaying the proportion of different categories within a whole. They are effective for visualizing the relative contributions of different components to a total. However, they are less suitable for comparing precise values or subtle differences.
Example: Showing the percentage composition of different components in a mixture.
6. Box Plots:
Box plots (also known as box-and-whisker plots) are excellent for summarizing the distribution of data, particularly when comparing distributions across multiple groups. They show the median, quartiles, and potential outliers of a dataset, offering a concise visual representation of data variability and central tendency.
Example: Comparing the variability of blood pressure measurements in different age groups.
Essential Elements of a Well-Constructed Graph
Regardless of the graph type chosen, several key elements contribute to creating a clear, informative, and scientifically rigorous graphical representation:
1. Clear and Concise Title:
The title should accurately reflect the content of the graph and clearly indicate the variables being represented.
2. Appropriately Labeled Axes:
Axes should be clearly labeled with the name of the variable and its units (e.g., "Time (seconds)", "Absorbance (AU)", "Concentration (µM)").
3. Appropriate Scale:
The scale of the axes should be chosen to accurately represent the range of data and facilitate easy interpretation. Avoid unnecessarily compressed or stretched scales.
4. Legend (if applicable):
If multiple datasets are plotted on the same graph, a clear legend is essential to distinguish the different data series.
5. Data Points and Error Bars (if applicable):
Data points should be clearly visible, and error bars (representing the uncertainty in the measurements) should be included when appropriate to indicate the precision of the data.
6. Appropriate Graph Type:
Selecting the correct graph type is crucial for accurately representing the data and avoiding misinterpretations.
7. Neat and Professional Appearance:
The graph should be aesthetically pleasing and easy to read. Avoid cluttered or confusing designs.
Avoiding Common Mistakes in Graph Construction
Several common pitfalls can compromise the clarity and accuracy of graphs. Avoiding these mistakes is vital for ensuring that your graphs effectively communicate your research findings:
- Overcrowding: Too much data on a single graph can make it difficult to interpret. Consider using multiple graphs to present complex data sets.
- Poorly Chosen Scales: Inappropriate scaling can distort the appearance of trends and relationships.
- Lack of Error Bars: Omitting error bars can overstate the precision of the data.
- Unclear Legends: Vague or missing legends can lead to confusion.
- Inconsistent Formatting: Inconsistent font sizes, colors, and styles can detract from the professionalism of the graph.
- Misleading Labels: Inaccurate or misleading labels can misrepresent the data.
- Incorrect Graph Type: Using the wrong type of graph can misrepresent the data and its relationships.
Software Tools for Creating Graphs
Several software packages are available for creating high-quality graphs for scientific purposes:
- Microsoft Excel: A widely accessible and versatile program for creating various graph types.
- GraphPad Prism: A specialized program for scientific graphing and data analysis.
- OriginPro: A powerful and flexible program for advanced scientific graphing and data analysis.
- MATLAB: A high-level programming language and interactive environment that is extensively used for scientific computing and graphing.
- R: An open-source statistical computing language with powerful graphing capabilities.
Conclusion
Graphs are invaluable tools in laboratory investigations, transforming raw data into visually compelling representations that reveal patterns, trends, and relationships. By carefully selecting the appropriate graph type and adhering to best practices for graph construction, researchers can effectively communicate their findings, support their scientific arguments, and advance their understanding of the phenomena under investigation. Mastering the art of scientific graphing is essential for any scientist or researcher. The ability to effectively visualize and interpret data is paramount to the success of any laboratory investigation. By utilizing the guidelines and best practices discussed in this article, you can significantly enhance the clarity, impact, and scientific rigor of your research.
Latest Posts
Latest Posts
-
How Do You Get Vaseline Out Of Carpet
May 11, 2025
-
12 Out Of 25 As A Grade
May 11, 2025
-
Compare Interstitial And Vacancy Atomic Mechanisms For Diffusion
May 11, 2025
-
What Is The Gcf Of 6 And 14
May 11, 2025
-
The Square Root Of 27 Divided By 3 Is
May 11, 2025
Related Post
Thank you for visiting our website which covers about Graphs Of The Data From Laboratory Investigations Are Used To . We hope the information provided has been useful to you. Feel free to contact us if you have any questions or need further assistance. See you next time and don't miss to bookmark.