The Information Gathered During An Experiment Is Called
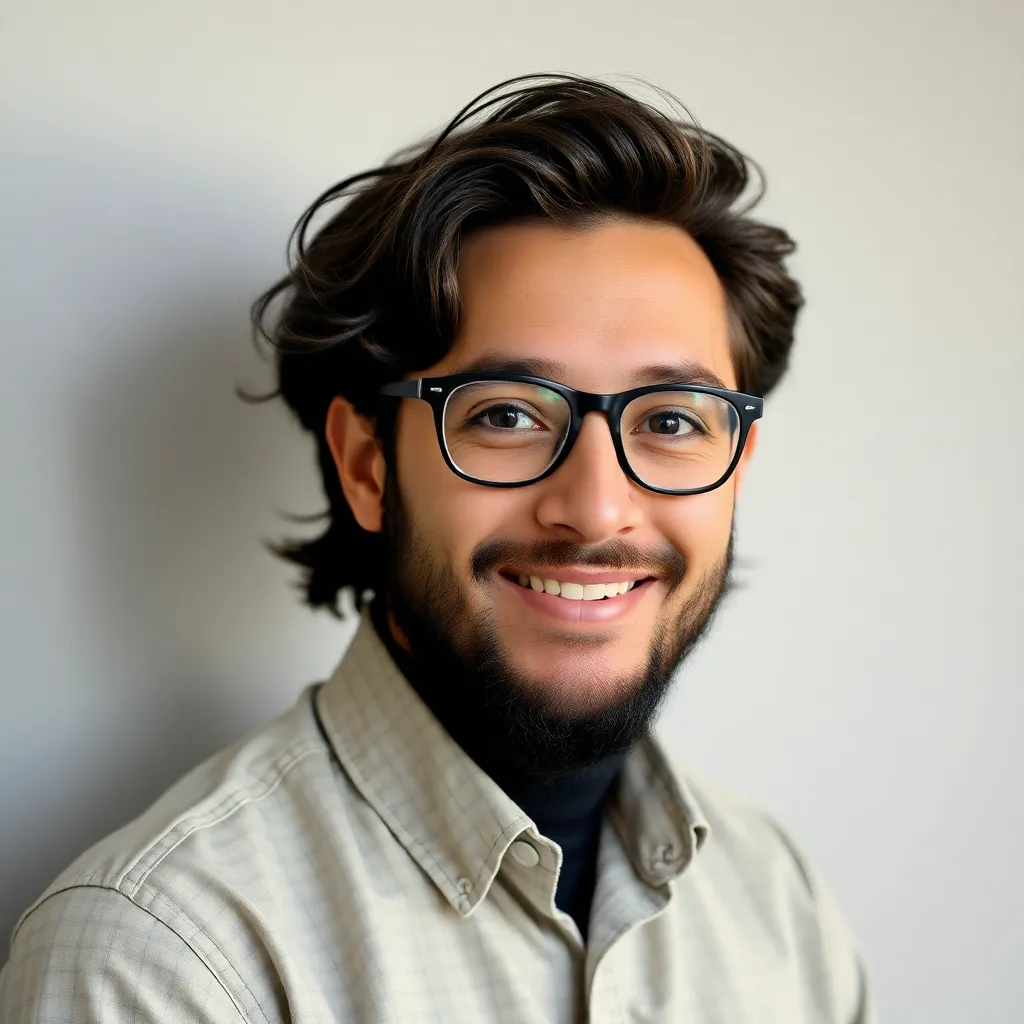
Arias News
May 10, 2025 · 6 min read
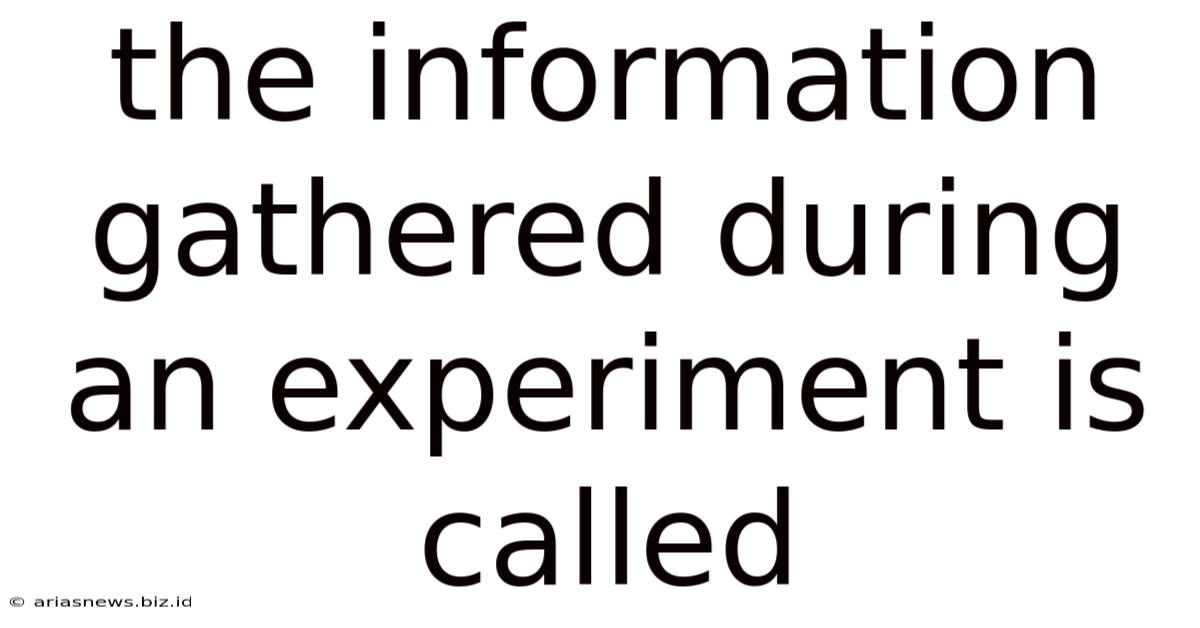
Table of Contents
The Information Gathered During an Experiment is Called: Data, and How to Make it Sing
The bedrock of any scientific endeavor, from the simplest classroom experiment to the most complex research project, lies in the data it generates. But what exactly is data in the context of an experiment, and how do we transform this raw information into meaningful insights? This article will delve into the multifaceted nature of experimental data, exploring its types, collection methods, analysis techniques, and ultimately, its crucial role in drawing conclusions and advancing knowledge.
Understanding Experimental Data: More Than Just Numbers
The information gathered during an experiment, broadly speaking, is called data. However, this simple definition belies the rich complexity of experimental data. It's not just a collection of numbers or observations; it's the raw material from which scientific understanding is forged. Data can take many forms, each requiring specific methods for collection, analysis, and interpretation.
Types of Experimental Data: A Diverse Landscape
Experimental data can be broadly categorized into several types:
-
Qualitative Data: This type of data describes qualities or characteristics. It's often descriptive and non-numerical, focusing on observations and interpretations. Examples include: color changes, texture descriptions, behavioral observations (e.g., animal activity), and interview transcripts. Qualitative data is valuable for understanding context and nuances that numerical data might miss. Analyzing qualitative data often involves techniques like thematic analysis and coding.
-
Quantitative Data: This involves numerical measurements and counts. It's often expressed as numbers, allowing for statistical analysis and precise comparisons. Examples include: height, weight, temperature, reaction rates, and survey responses (e.g., number of people choosing a specific option). Quantitative data provides objective measurements and facilitates robust statistical analysis.
-
Discrete Data: This type of quantitative data represents counts that can only take on specific, separate values. For example, the number of heads obtained when flipping a coin (0, 1, 2, etc.) or the number of students in a classroom. Discrete data cannot be meaningfully broken down into smaller units.
-
Continuous Data: This type of quantitative data can take on any value within a given range. Examples include: height (can be measured to any degree of precision), temperature, weight, and time. Continuous data can be further subdivided and analyzed across a spectrum of values.
-
Primary Data: This refers to data collected directly by the researcher during the experiment. It's firsthand information gathered through direct observation, experimentation, or surveys designed specifically for the research. Primary data is often considered the most reliable as it's not subject to the biases or interpretations of others.
-
Secondary Data: This comprises data that already exists and has been collected by someone else. Examples include: published research papers, government statistics, and existing databases. While convenient and readily available, secondary data needs careful evaluation for its reliability and relevance to the current experiment.
Data Collection Methods: Precision and Accuracy are Key
The way data is collected significantly impacts its quality and reliability. The choice of data collection methods depends heavily on the nature of the experiment and the type of data being collected.
Common Data Collection Methods:
-
Observations: This involves systematically watching and recording events or behaviors. Structured observations use pre-defined categories and recording sheets, while unstructured observations allow for more flexibility but may be harder to analyze objectively.
-
Measurements: This is the process of using instruments to obtain numerical data. Accuracy and precision are crucial in measurement, with proper calibration and consideration of potential errors being essential.
-
Surveys: Surveys involve collecting data through questionnaires or interviews. Careful design is essential to avoid bias and ensure valid responses. Different survey types exist, including closed-ended (multiple choice) and open-ended (free-response) questions.
-
Experiments: Controlled experiments involve manipulating variables to observe their effects. Data is collected by measuring the outcomes of these manipulations. Careful consideration of experimental design is essential to minimize confounding variables and ensure reliable results.
-
Interviews: These involve direct conversations with participants to gather qualitative data. Structured interviews use standardized questions, while unstructured interviews allow for more flexible probing and deeper insights.
-
Focus Groups: These bring together a group of individuals to discuss a particular topic, providing insights into shared opinions and perspectives.
Data Analysis: Transforming Raw Data into Meaningful Insights
Once the data is collected, it needs to be meticulously analyzed to extract meaningful insights. This process often involves several steps:
Data Cleaning and Preparation:
Before any analysis can begin, the data needs to be cleaned and prepared. This involves:
- Handling missing data: This can involve imputation (estimating missing values) or exclusion of incomplete data sets.
- Identifying and correcting errors: Errors can arise from data entry mistakes or instrument malfunctions.
- Data transformation: This may involve changing the format or scale of the data to make it suitable for analysis. For instance, converting data to a standardized score (z-score) for comparison across different datasets.
Descriptive Statistics: Summarizing Data:
Descriptive statistics provide a summary of the data's key characteristics. Common descriptive statistics include:
- Mean: The average value.
- Median: The middle value when the data is ordered.
- Mode: The most frequent value.
- Standard deviation: A measure of the data's spread or variability.
- Range: The difference between the highest and lowest values.
Graphs and charts (histograms, bar charts, scatter plots) are often used to visually represent the data and its distribution.
Inferential Statistics: Drawing Conclusions from Data:
Inferential statistics allow researchers to draw conclusions about a population based on a sample of data. Common inferential statistical tests include:
- t-tests: Used to compare the means of two groups.
- ANOVA (Analysis of Variance): Used to compare the means of three or more groups.
- Correlation analysis: Used to determine the strength and direction of the relationship between two variables.
- Regression analysis: Used to model the relationship between a dependent variable and one or more independent variables.
- Chi-square test: Used to analyze categorical data and assess the association between variables.
Ensuring Data Integrity: Ethical Considerations and Best Practices
Maintaining the integrity of experimental data is paramount. This involves adhering to ethical guidelines and employing best practices throughout the research process.
Ethical Considerations:
- Informed consent: Participants must be fully informed about the study's purpose, procedures, and potential risks before participating.
- Confidentiality: Participant data should be kept confidential and protected from unauthorized access.
- Data security: Data should be stored securely to prevent loss or unauthorized access.
- Data transparency: Research findings should be reported honestly and transparently, including any limitations of the study.
Best Practices for Data Management:
- Detailed record keeping: Maintain meticulous records of all aspects of the experiment, including data collection methods, analysis techniques, and any limitations.
- Data version control: Track changes made to the data to ensure reproducibility and traceability.
- Data backup and redundancy: Regularly back up data to prevent loss due to hardware failure or other unforeseen events.
- Data sharing: Consider sharing data with other researchers (where appropriate) to promote transparency and collaboration.
The Power of Data: Driving Scientific Advancements
The information gathered during an experiment—the data—is far more than just numbers or observations; it's the engine that drives scientific discovery. By employing rigorous data collection methods, careful analysis techniques, and ethical considerations, researchers can transform raw data into meaningful insights, leading to advancements in our understanding of the world. The journey from data collection to robust conclusions requires precision, critical thinking, and a deep understanding of statistical principles. The data itself might be silent, but with proper handling and analysis, it speaks volumes, revealing the secrets of the natural world and propelling scientific progress. The meticulous handling and interpretation of this data are the cornerstones of advancing scientific knowledge and shaping our future.
Latest Posts
Latest Posts
-
1989 Chevy Silverado Interior Door Pull Strap
May 11, 2025
-
Distractions And Driver Inattention Are A Cause Of 65
May 11, 2025
-
How Many Square Feet Is 46 Acres
May 11, 2025
-
What Is 1 1 4 Cup Doubled In Cooking
May 11, 2025
-
Is Freezing A Chemical Or Physical Change
May 11, 2025
Related Post
Thank you for visiting our website which covers about The Information Gathered During An Experiment Is Called . We hope the information provided has been useful to you. Feel free to contact us if you have any questions or need further assistance. See you next time and don't miss to bookmark.