The Ll Theorem Is A Special Case Of The
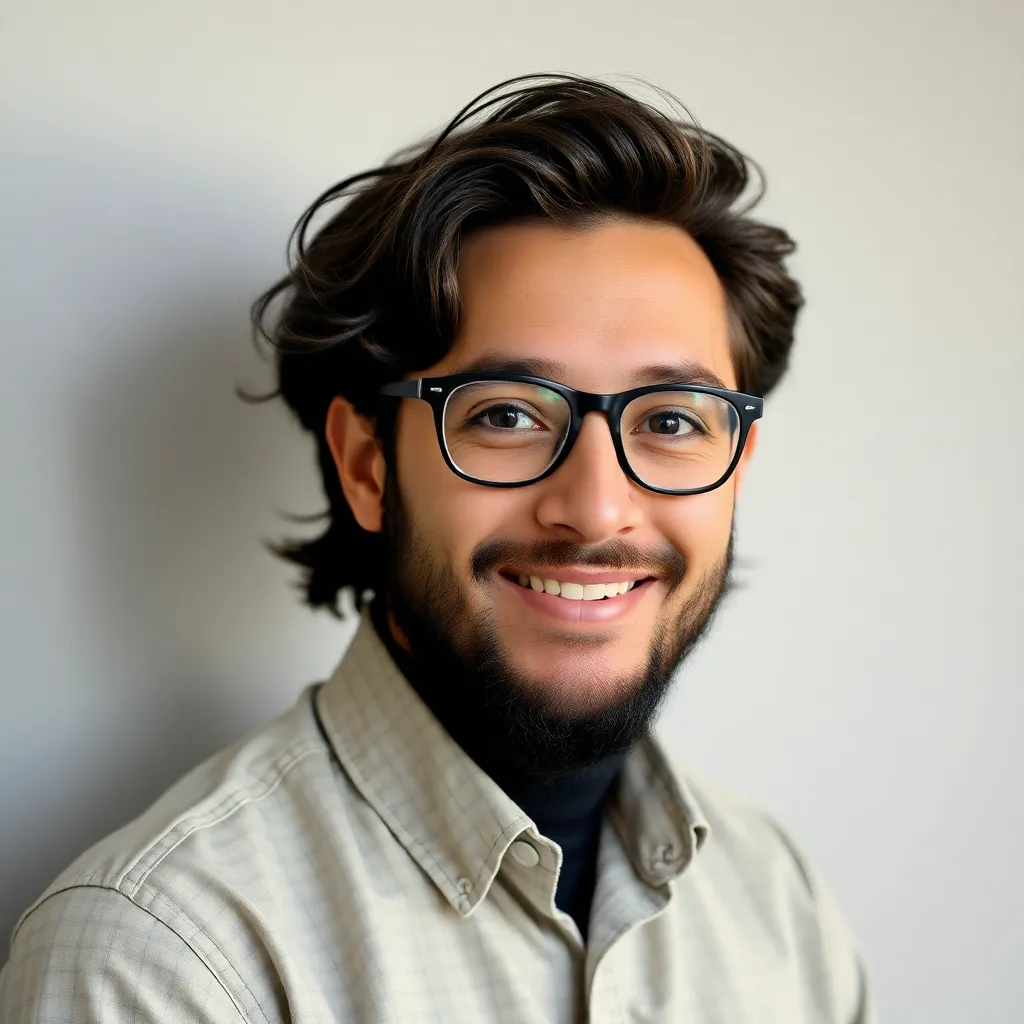
Arias News
Apr 14, 2025 · 5 min read

Table of Contents
The Law of Large Numbers: A Special Case of the Central Limit Theorem?
The Law of Large Numbers (LLN) and the Central Limit Theorem (CLT) are two cornerstones of probability theory and statistics, both dealing with the behavior of sums of random variables. While seemingly distinct, a deeper understanding reveals a fascinating relationship: the Law of Large Numbers can be viewed as a special case of the Central Limit Theorem, albeit a somewhat degenerate one. This article will explore this relationship, examining both theorems individually before delving into the connection and its implications.
Understanding the Law of Large Numbers (LLN)
The Law of Large Numbers states that as the number of independent and identically distributed (i.i.d.) random variables increases, the sample average converges towards the expected value (mean) of the population. There are two main versions:
1. Weak Law of Large Numbers (WLLN)
The WLLN states that the sample average converges in probability to the expected value. In simpler terms, the probability that the sample average deviates significantly from the expected value becomes increasingly small as the sample size increases. Mathematically, this is expressed as:
P(|X̄ - μ| > ε) → 0 as n → ∞
Where:
- X̄ is the sample average
- μ is the population mean
- ε is an arbitrarily small positive number
- n is the sample size
2. Strong Law of Large Numbers (SLLN)
The SLLN is a stronger statement, asserting that the sample average converges almost surely to the expected value. This means that the probability that the sample average eventually settles down to the expected value is 1. Formally:
P(lim<sub>n→∞</sub> X̄ = μ) = 1
The SLLN implies the WLLN, but not vice versa. The SLLN provides a more robust guarantee of convergence. Both versions, however, highlight the fundamental idea: the sample average becomes a reliable estimator of the population mean as the sample size grows.
Deconstructing the Central Limit Theorem (CLT)
The Central Limit Theorem is significantly more powerful and nuanced than the LLN. It describes not only the convergence of the sample average but also the distribution of the sample average as the sample size increases.
The CLT states that the distribution of the sample average, properly normalized, converges to a standard normal distribution (mean 0, variance 1) regardless of the distribution of the individual random variables (provided they have a finite mean and variance). More precisely:
Z = (X̄ - μ) / (σ / √n) → N(0,1) as n → ∞
Where:
- Z is the standardized sample average
- σ is the population standard deviation
- N(0,1) represents the standard normal distribution
This is a remarkably powerful statement. It means that even if the original data is highly skewed or non-normal, the distribution of the sample average will approach a bell curve as the sample size increases. This forms the basis for many statistical tests and confidence intervals.
The LLN as a Degenerate Case of the CLT
The connection between the LLN and the CLT lies in the convergence of the standardized sample average. Consider the CLT formula again:
Z = (X̄ - μ) / (σ / √n)
As n approaches infinity, the denominator (σ / √n) approaches zero. This means the standardized variable Z will tend towards infinity or negative infinity if (X̄ - μ) is not zero. However, the LLN tells us that (X̄ - μ) will converge to zero.
Therefore, although the CLT describes the distribution of the standardized sample average converging to a normal distribution, this is, in essence, a degenerate normal distribution – a distribution which collapses into a point at zero. This is because the denominator shrinks in a way that ensures (X̄ - μ) must also approach zero to maintain any finite Z. The convergence to zero represents the convergence of the sample average to the population mean, which is precisely the essence of the LLN.
In a way, the CLT provides a more comprehensive picture; it explains not just the convergence of the average (the LLN's focus) but also the manner in which this convergence occurs, governed by the spreading of the distribution as related to the standard error (σ/√n). As sample size increases, the standard error decreases, leading the distribution to collapse onto the population mean. This collapse is the LLN.
Think of it this way: the CLT describes a continuously shrinking bell curve centered on the population mean. As the sample size grows infinitely large, the bell curve's width shrinks to zero, ultimately leaving only a point mass at the population mean. This point mass represents the deterministic convergence predicted by the LLN.
Implications and Further Considerations
The relationship between the LLN and CLT highlights the profound implications of increasing sample size in statistical inference. The LLN provides the assurance of accurate estimation, while the CLT adds precision by characterizing the distribution of this estimation error.
However, it's crucial to remember that both theorems rely on the assumption of independent and identically distributed random variables. Violations of these assumptions can significantly impact the applicability of both the LLN and CLT. For example, in time series data where autocorrelation exists, the LLN and CLT might not hold directly, necessitating more advanced statistical techniques.
Furthermore, the rate of convergence in both theorems is crucial. While the LLN guarantees convergence, it doesn't specify how quickly this happens. Similarly, the CLT's convergence to the normal distribution is asymptotic – it happens as n approaches infinity. In practice, we deal with finite sample sizes, and understanding the rate of convergence helps us assess the reliability of our estimates.
Conclusion
The Law of Large Numbers and the Central Limit Theorem are fundamental theorems in probability and statistics. Although distinct, their relationship is profound. The LLN can be seen as a special, degenerate case of the CLT, where the variance of the distribution collapses to zero as the sample size grows, resulting in the convergence of the sample mean to the population mean. This insight deepens our understanding of the power of large sample sizes and the behavior of statistical estimators. The CLT offers a more comprehensive description than the LLN. It tells us not only that we get closer to the true mean, but that the approximation asymptotically follows a normal distribution. This is crucial for building confidence intervals and conducting hypothesis testing. Ultimately, both theorems underpin a substantial portion of modern statistical practice.
Latest Posts
Latest Posts
-
I Look Forward To Meeting You Soon
Apr 15, 2025
-
How Tall Is 1 56 Meters In Feet
Apr 15, 2025
-
How Many Cups Of Sugar In 4 Lbs
Apr 15, 2025
-
How Big Is Italy Compared To The Us
Apr 15, 2025
-
When Does Stefan Tell Elena Hes A Vampire
Apr 15, 2025
Related Post
Thank you for visiting our website which covers about The Ll Theorem Is A Special Case Of The . We hope the information provided has been useful to you. Feel free to contact us if you have any questions or need further assistance. See you next time and don't miss to bookmark.