Two Events Are Said To Be Correlated If
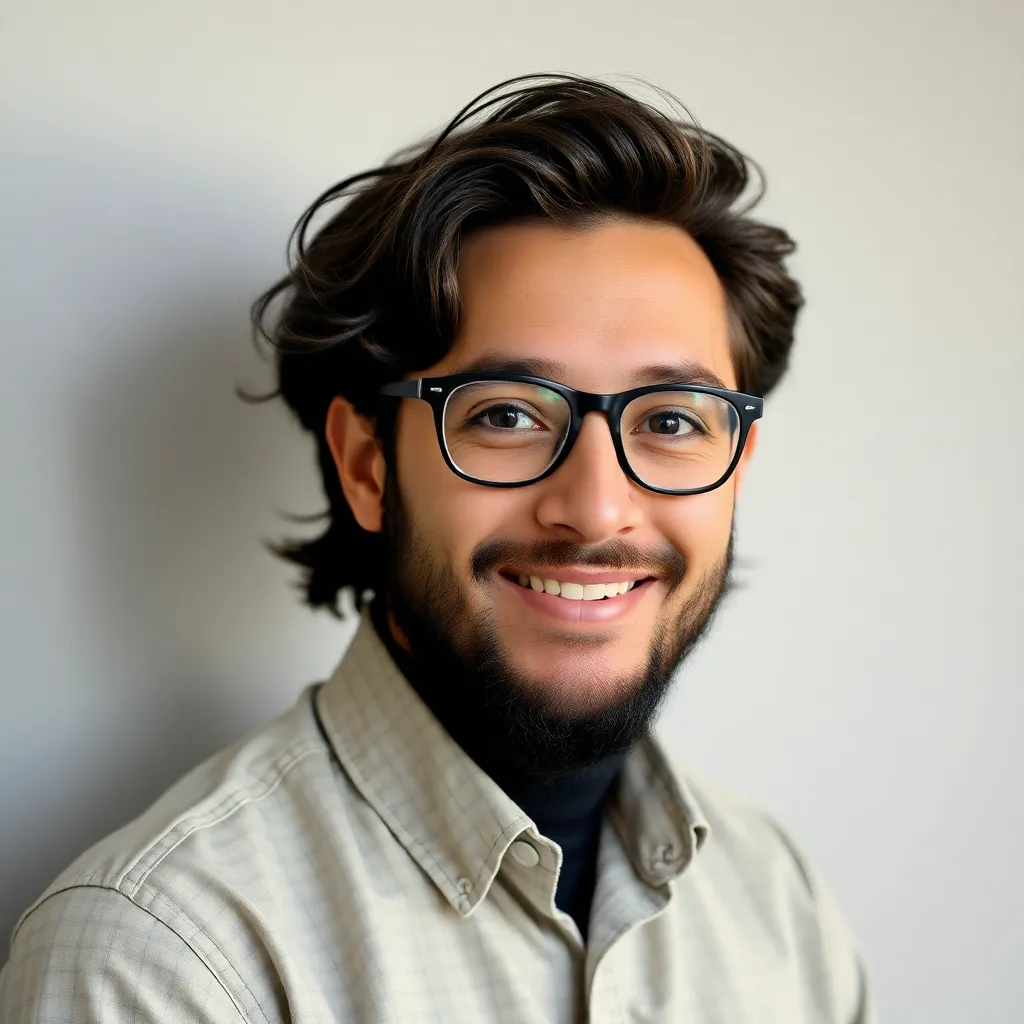
Arias News
May 12, 2025 · 6 min read
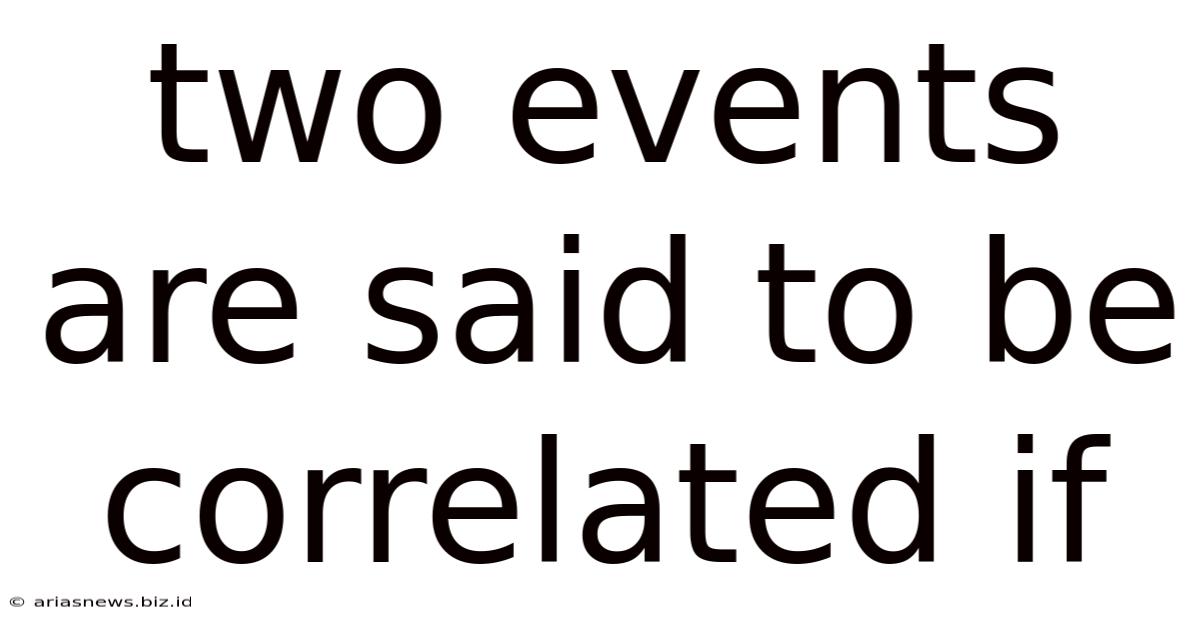
Table of Contents
Two Events Are Said to Be Correlated If... Understanding Correlation in Statistics
Correlation is a fundamental concept in statistics that describes the relationship between two or more variables. Understanding correlation is crucial in numerous fields, from finance and healthcare to social sciences and environmental studies. This article delves deep into the meaning of correlation, exploring different types of correlation, how to interpret correlation coefficients, and the crucial distinction between correlation and causation.
What Does it Mean When Two Events Are Correlated?
Two events are said to be correlated if a change in one variable is associated with a change in another variable. This association can be positive, negative, or even non-existent. A positive correlation means that as one variable increases, the other variable tends to increase as well. A negative correlation implies that as one variable increases, the other tends to decrease. No correlation indicates that there's no discernible relationship between the two variables.
It's crucial to understand that correlation does not equal causation. Just because two events are correlated doesn't automatically mean that one causes the other. There might be a third, unseen variable influencing both, or the relationship could be purely coincidental.
Types of Correlation
While the basic definition outlines positive, negative, and no correlation, the nuances of correlation are more complex. We can categorize correlation in several ways:
1. Linear vs. Non-linear Correlation
-
Linear Correlation: This is the most common type, where the relationship between the two variables can be represented by a straight line. As one variable increases, the other increases or decreases at a relatively constant rate. This is what's typically measured by the Pearson correlation coefficient.
-
Non-linear Correlation: In this case, the relationship between the variables isn't linear. The relationship might be curvilinear (e.g., an inverted U-shape) or follow a more complex pattern. Linear correlation coefficients are not suitable for assessing non-linear relationships. Methods like Spearman's rank correlation might be more appropriate.
2. Positive, Negative, and Zero Correlation
-
Positive Correlation: As mentioned earlier, a positive correlation exists when an increase in one variable is associated with an increase in the other. Examples include height and weight (taller people tend to weigh more), or study time and exam scores (more study time often leads to better scores). The correlation coefficient will be positive, ranging from 0 to +1.
-
Negative Correlation: A negative correlation occurs when an increase in one variable is associated with a decrease in the other. For instance, the relationship between hours spent watching TV and exam scores might be negatively correlated (more TV time, lower scores). The correlation coefficient will be negative, ranging from -1 to 0.
-
Zero Correlation: This means there's no linear relationship between the variables. Changes in one variable don't seem to predict changes in the other. However, this doesn't necessarily imply that there's no relationship at all; it simply means there's no linear relationship. A zero correlation coefficient doesn't rule out the possibility of a non-linear relationship.
3. Pearson, Spearman, and Kendall Correlation
Different correlation coefficients are used depending on the type of data and the nature of the relationship:
-
Pearson Correlation Coefficient (r): This is the most commonly used measure for linear correlation between two continuous variables. It measures the strength and direction of the linear relationship. The value of 'r' ranges from -1 to +1. A value of +1 indicates a perfect positive linear correlation, -1 indicates a perfect negative linear correlation, and 0 indicates no linear correlation.
-
Spearman's Rank Correlation Coefficient (ρ): This is a non-parametric measure of correlation that assesses the monotonic relationship between two variables. It's used when the data doesn't meet the assumptions of Pearson correlation (e.g., data isn't normally distributed). Spearman's correlation uses ranks instead of the actual data values.
-
Kendall's Tau (τ): Another non-parametric measure of rank correlation, Kendall's tau is often preferred over Spearman's rho when dealing with small datasets or a large number of tied ranks. It measures the ordinal association between two measured quantities.
Interpreting Correlation Coefficients
Interpreting correlation coefficients requires understanding the magnitude and sign of the coefficient:
-
Magnitude: The absolute value of the correlation coefficient indicates the strength of the relationship. A value close to 1 (either positive or negative) indicates a strong relationship, while a value close to 0 indicates a weak relationship. A common interpretation is:
- |r| < 0.3: Weak correlation
- 0.3 ≤ |r| < 0.7: Moderate correlation
- |r| ≥ 0.7: Strong correlation
-
Sign: The sign of the coefficient (+ or -) indicates the direction of the relationship (positive or negative).
Correlation vs. Causation: A Critical Distinction
The most crucial point to remember about correlation is that correlation does not imply causation. Even a strong correlation between two variables doesn't prove that one causes the other. Several reasons explain this:
-
Third Variable Problem (Confounding Variable): An unobserved variable might be influencing both variables, creating a spurious correlation. For example, ice cream sales and drowning incidents are positively correlated, but this doesn't mean ice cream causes drowning. The confounding variable is hot weather: both ice cream sales and swimming increase during hot weather.
-
Reverse Causation: The causal relationship might be the opposite of what's initially assumed. For example, while higher education might be correlated with higher income, it's not necessarily the case that higher education causes higher income. People with higher innate abilities might be more likely to pursue higher education and achieve higher incomes.
-
Coincidence: Sometimes, a correlation is simply due to chance, especially with small sample sizes.
Applications of Correlation Analysis
Correlation analysis is a powerful tool with wide-ranging applications across various fields:
-
Finance: Analyzing the correlation between different assets to build diversified investment portfolios.
-
Healthcare: Studying the correlation between lifestyle factors and disease risk.
-
Social Sciences: Investigating the correlation between social factors and various outcomes (e.g., education levels and crime rates).
-
Environmental Science: Analyzing the correlation between pollution levels and environmental damage.
-
Marketing: Understanding the correlation between marketing campaigns and sales.
Limitations of Correlation Analysis
While correlation analysis is invaluable, it has limitations:
-
Non-linear relationships: Correlation coefficients (like Pearson's r) are primarily designed for linear relationships. Non-linear relationships might be missed.
-
Outliers: Extreme values (outliers) can significantly influence the correlation coefficient.
-
Causation: As emphasized repeatedly, correlation doesn't imply causation.
Conclusion: Understanding the Nuances of Correlation
Understanding correlation is crucial for interpreting data accurately and making informed decisions. This article has explored the different types of correlation, methods for measuring correlation, and the critical distinction between correlation and causation. By carefully considering the type of data, selecting the appropriate correlation coefficient, and interpreting results cautiously, researchers can use correlation analysis to uncover valuable insights into the relationships between variables. Always remember to consider potential confounding variables and avoid drawing causal conclusions based solely on correlation. Further investigation, potentially using experimental designs, is often needed to establish causality. Proper understanding and application of correlation analysis are essential for sound statistical reasoning and informed decision-making across many disciplines.
Latest Posts
Latest Posts
-
How Much Is An Eighth Of An Inch
May 12, 2025
-
A Trapezoid Has Two Pairs Of Parallel Sides
May 12, 2025
-
When Manually Cleaning Equipment In A Three Compartment Sink
May 12, 2025
-
What Is The First 5 Multiples Of 4
May 12, 2025
-
How Did The Caddo Get Their Food
May 12, 2025
Related Post
Thank you for visiting our website which covers about Two Events Are Said To Be Correlated If . We hope the information provided has been useful to you. Feel free to contact us if you have any questions or need further assistance. See you next time and don't miss to bookmark.