What Does It Mean If A Scale Is Linear
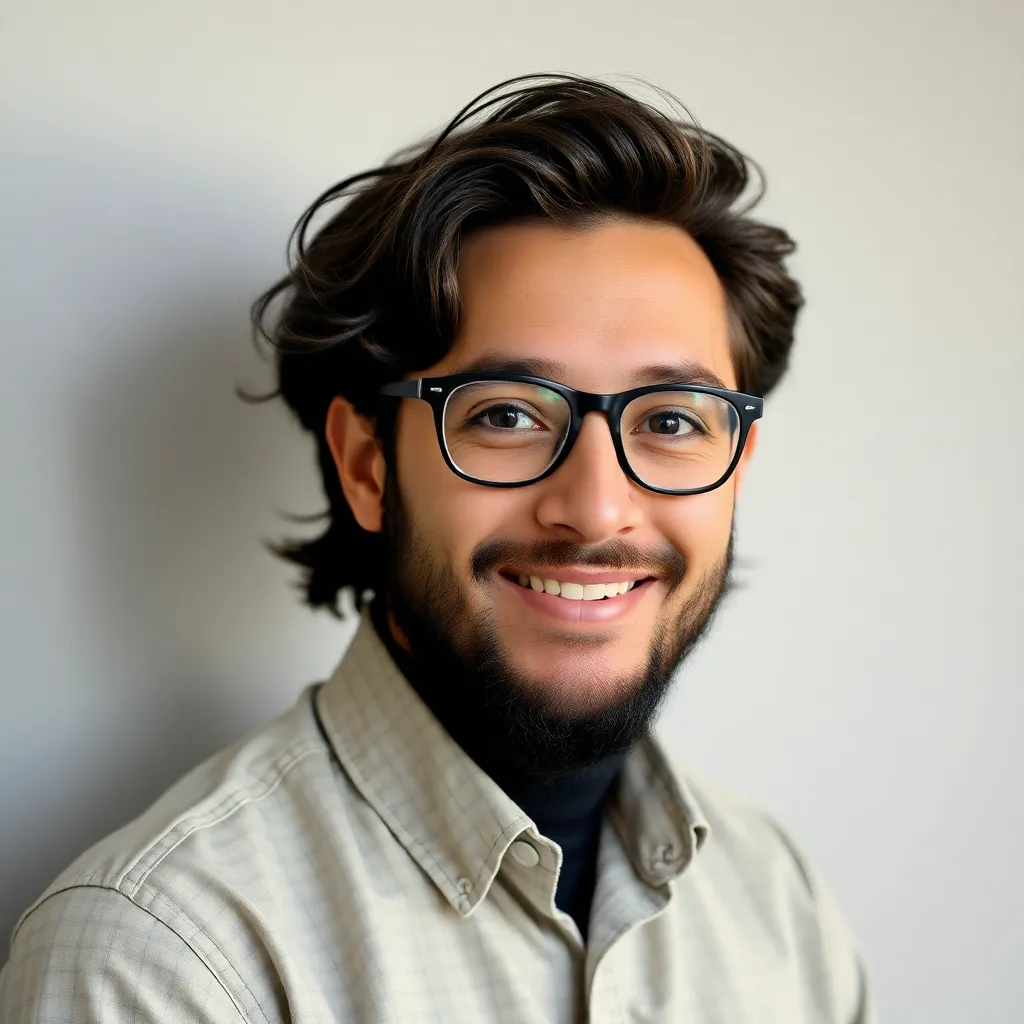
Arias News
Apr 25, 2025 · 6 min read

Table of Contents
What Does It Mean If a Scale is Linear? A Deep Dive into Linearity
Understanding linearity is crucial in various fields, from mathematics and physics to data science and everyday life. A linear scale, at its core, represents a consistent, proportional relationship between two variables. This means that a change in one variable results in a directly proportional change in the other. This article will explore the concept of linearity in detail, covering its mathematical definition, real-world applications, and the distinctions between linear and non-linear scales. We'll also delve into how to identify linearity in data and its implications for analysis and interpretation.
Defining Linearity: The Mathematical Perspective
Mathematically, linearity is defined by two key properties:
1. Additivity: If we have a function f(x), it's additive if f(x + y) = f(x) + f(y) for all values of x and y. This means that the function's output for the sum of two inputs is equal to the sum of the function's outputs for each input individually.
2. Homogeneity: A function is homogeneous if f(cx) = cf(x) for any constant c. This implies that scaling the input by a constant factor scales the output by the same factor.
A function that satisfies both additivity and homogeneity is considered a linear function. The simplest example is a straight line represented by the equation y = mx + c, where 'm' is the slope (representing the rate of change) and 'c' is the y-intercept (the value of y when x is 0).
Visualizing Linearity: The Straight Line
The most intuitive representation of a linear scale is a straight line on a graph. The slope of this line signifies the constant rate of change between the two variables. A steeper slope indicates a faster rate of change, while a flatter slope indicates a slower rate. A horizontal line (slope = 0) represents no change in the dependent variable regardless of the independent variable.
Examples of Linear Scales in Everyday Life
Linear scales are prevalent in many everyday situations:
- Temperature scales (Celsius and Fahrenheit): While the conversion between Celsius and Fahrenheit isn't strictly additive, within each scale itself, a change of 1 degree represents a consistent change in temperature.
- Speedometer readings: A speedometer displays a linear relationship between speed and distance covered over time, assuming constant speed.
- Mapping and scaling: Maps often use linear scales to represent geographical distances, allowing for accurate measurements of distances between locations.
- Simple interest calculations: Simple interest calculations involve a linear relationship between the principal amount, interest rate, and time.
- Direct Proportionality: Any scenario exhibiting a direct proportional relationship between two variables can be represented by a linear scale. For instance, the cost of purchasing multiple identical items is directly proportional to the number of items purchased.
Non-Linear Scales: Understanding the Differences
It's equally important to understand what constitutes a non-linear scale. Non-linear scales represent relationships where a change in one variable doesn't result in a directly proportional change in the other. These relationships are often more complex and can be represented by curves rather than straight lines on a graph.
Examples of Non-Linear Scales
Many real-world phenomena exhibit non-linear relationships:
- Exponential growth: Population growth, compound interest, and the spread of viruses often follow exponential patterns, characterized by rapidly accelerating growth.
- Decay processes: Radioactive decay and the cooling of an object follow exponential decay patterns, where the rate of change decreases over time.
- Logistic growth: This model describes growth that initially increases rapidly but eventually levels off due to limiting factors. Examples include the spread of a rumor or the adoption of a new technology.
- Power law relationships: Many physical phenomena, such as the intensity of light from a source or the gravitational force between two objects, follow power law relationships, where one variable is proportional to a power of the other.
Identifying Linearity in Data: Practical Approaches
Identifying linearity in data is crucial for applying appropriate statistical methods and drawing accurate conclusions. Several methods can be employed:
1. Visual Inspection: Plotting the data on a scatter plot provides the simplest way to assess linearity. If the data points roughly align along a straight line, it suggests a linear relationship.
2. Correlation Coefficient: The Pearson correlation coefficient (r) measures the linear association between two variables. A value of +1 indicates a perfect positive linear correlation, -1 indicates a perfect negative linear correlation, and 0 indicates no linear correlation. However, a high correlation doesn't necessarily imply causality.
3. Regression Analysis: Linear regression models attempt to fit a straight line through the data points. The goodness of fit can be assessed using metrics like R-squared, which represents the proportion of variance in the dependent variable explained by the independent variable. A high R-squared value suggests a good fit.
4. Residual Analysis: Analyzing the residuals (differences between observed and predicted values) can reveal deviations from linearity. If the residuals show patterns or trends, it indicates a non-linear relationship.
Implications of Linearity in Data Analysis
The presence or absence of linearity significantly impacts the choice of statistical methods and the interpretation of results. Linear models are easier to interpret and computationally less demanding than non-linear models. However, forcing a linear model on non-linear data can lead to inaccurate conclusions and misleading predictions.
Linearity in Different Fields
The concept of linearity finds applications in diverse fields:
1. Physics: Many fundamental laws of physics, such as Newton's second law of motion (F=ma), are linear. However, many real-world phenomena deviate from strict linearity, especially at extreme scales or conditions.
2. Engineering: Linear systems are easier to design, analyze, and control. Linear algebra is a fundamental tool in structural analysis, circuit design, and signal processing.
3. Economics: Linear models are widely used in econometrics to model economic relationships. However, economic behavior is often complex and non-linear.
4. Computer Science: Linear algebra is essential in computer graphics, machine learning, and data mining. Linear programming is a powerful optimization technique with applications in resource allocation and scheduling.
5. Machine Learning: Linear regression is a fundamental algorithm in machine learning, used for prediction and classification. However, more complex non-linear models, such as support vector machines (SVMs) and neural networks, are often needed to capture complex relationships in data.
Conclusion: The Importance of Recognizing Linearity
Understanding the concept of linearity is essential for anyone working with data or models. Recognizing whether a relationship is linear or non-linear allows for the selection of appropriate analytical techniques, ensuring accurate interpretations and reliable predictions. While linear models offer simplicity and interpretability, recognizing the limitations of linearity and embracing non-linear approaches when necessary is crucial for capturing the complexity of the real world. By mastering the nuances of linearity, you can enhance your analytical skills and unlock a deeper understanding of the data you encounter. Remember to always carefully examine your data, consider the underlying processes, and choose the most appropriate model to represent the relationships accurately. Ignoring the nature of linearity can lead to inaccurate conclusions and poor decision-making.
Latest Posts
Latest Posts
-
How To Say Thank You Beautiful In Spanish
Apr 25, 2025
-
When Is National Give Your Gf A Hoodie Day
Apr 25, 2025
-
What Is The Application In A Science Project
Apr 25, 2025
-
5 Times The Sum Of 7 And 23
Apr 25, 2025
-
What Is A Polygon That Has 9 Sides
Apr 25, 2025
Related Post
Thank you for visiting our website which covers about What Does It Mean If A Scale Is Linear . We hope the information provided has been useful to you. Feel free to contact us if you have any questions or need further assistance. See you next time and don't miss to bookmark.