Which Of These R-values Represents The Strongest Correlation
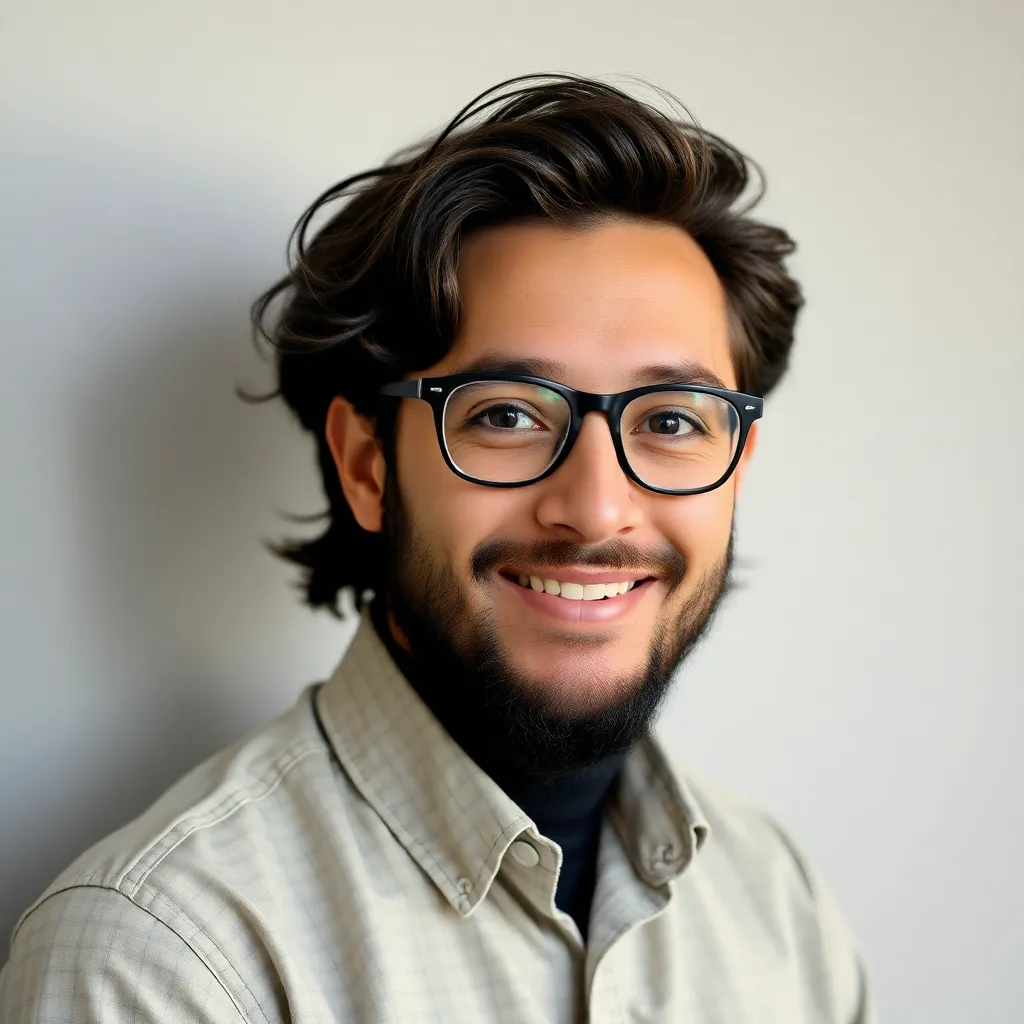
Arias News
May 11, 2025 · 5 min read
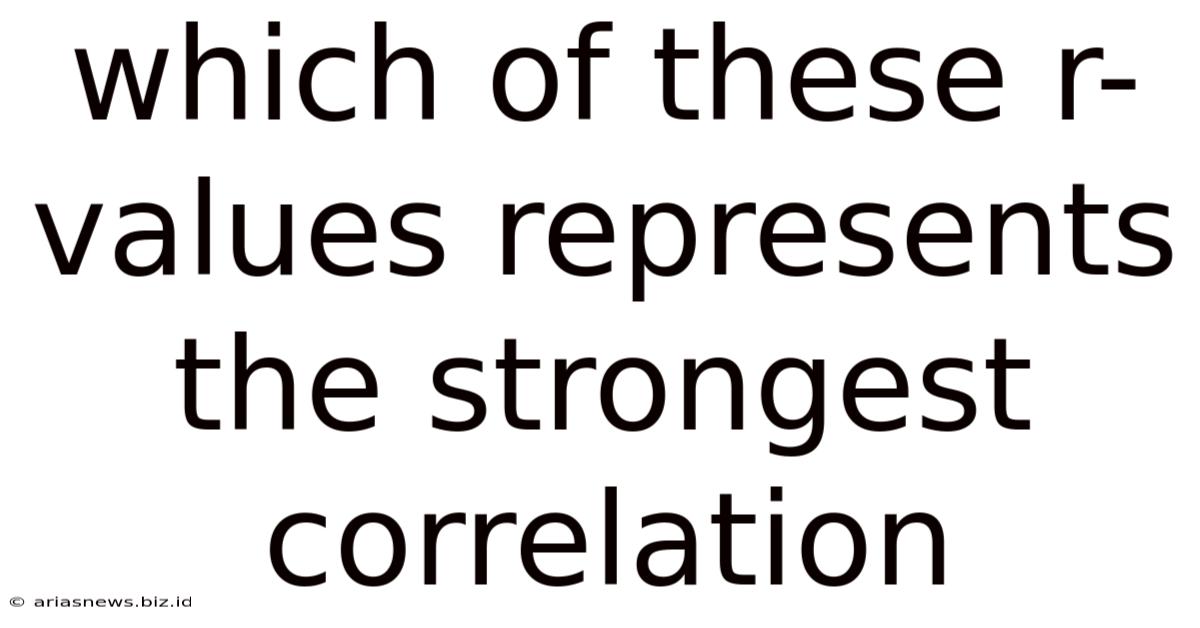
Table of Contents
Which of These R-Values Represents the Strongest Correlation? Understanding Correlation Coefficients
Correlation is a fundamental concept in statistics that quantifies the strength and direction of a linear relationship between two variables. Understanding correlation, and specifically the correlation coefficient (often represented by 'r'), is crucial for interpreting data and making informed decisions across various fields, from finance and healthcare to social sciences and engineering. This article delves deep into the interpretation of the correlation coefficient, helping you understand which r-values represent the strongest correlation.
Understanding the Correlation Coefficient (r)
The correlation coefficient, 'r', is a standardized measure ranging from -1 to +1. This value indicates both the strength and direction of the linear relationship:
-
Strength: The absolute value of 'r' represents the strength of the correlation. A value closer to 1 (positive or negative) signifies a stronger relationship, while a value closer to 0 indicates a weaker relationship.
-
Direction: The sign of 'r' indicates the direction of the relationship:
- Positive (r > 0): As one variable increases, the other tends to increase. This represents a positive correlation.
- Negative (r < 0): As one variable increases, the other tends to decrease. This represents a negative correlation.
- Zero (r = 0): There is no linear relationship between the variables. Note that this doesn't necessarily mean there's no relationship at all; it simply means there's no linear relationship. A non-linear relationship could exist.
Interpreting Different R-Values: A Detailed Guide
Let's explore how to interpret different r-values to determine the strength of correlation:
-
|r| = 1.0: This represents a perfect linear correlation. All data points lie exactly on a straight line. This is rare in real-world datasets.
-
0.8 ≤ |r| < 1.0: This indicates a very strong correlation. The data points cluster closely around a straight line. There's a high degree of association between the variables.
-
0.6 ≤ |r| < 0.8: This suggests a strong correlation. The data points show a clear linear trend, although there's more scatter than in a very strong correlation.
-
0.4 ≤ |r| < 0.6: This represents a moderate correlation. The linear trend is noticeable, but there's considerable scatter around the line.
-
0.2 ≤ |r| < 0.4: This indicates a weak correlation. The linear trend is weak, and there's substantial scatter. The relationship between the variables is barely discernible.
-
|r| < 0.2: This suggests a very weak or negligible correlation. There's little to no linear relationship between the variables. The scatter is substantial, and any observed trend is likely due to chance.
Example:
Consider these r-values: 0.95, -0.82, 0.30, -0.15, 0.70
To determine which represents the strongest correlation, we look at the absolute values:
- |0.95| = 0.95
- |-0.82| = 0.82
- |0.30| = 0.30
- |-0.15| = 0.15
- |0.70| = 0.70
Therefore, 0.95 represents the strongest correlation, followed by -0.82, 0.70, 0.30, and -0.15. The negative sign simply indicates an inverse relationship; it doesn't affect the strength of the correlation.
Factors Affecting Correlation: Beyond the R-Value
While the r-value provides a crucial measure of correlation, it's vital to consider other factors:
-
Causation vs. Correlation: A high correlation doesn't necessarily imply causation. Two variables might be strongly correlated due to a third, unobserved variable (confounding variable). For example, ice cream sales and crime rates might be positively correlated, but this doesn't mean ice cream causes crime. Both are likely influenced by a third variable: hot weather.
-
Outliers: Extreme values (outliers) can significantly influence the correlation coefficient. A single outlier can artificially inflate or deflate the r-value, misleading the interpretation of the relationship. Careful examination of the data for outliers is essential.
-
Non-linear Relationships: The correlation coefficient only measures linear relationships. If the relationship between variables is non-linear (e.g., quadratic, exponential), the r-value might be close to zero even if a strong relationship exists. Visual inspection of scatter plots is vital to identify non-linear relationships.
-
Sample Size: The reliability of the correlation coefficient increases with sample size. A small sample size might produce a high r-value due to random chance, while a larger sample size provides a more robust estimate of the true correlation.
-
Data Distribution: The assumption of a linear relationship is most valid when the data is approximately normally distributed. If the data is significantly skewed or has heavy tails, the interpretation of the r-value needs careful consideration.
Advanced Correlation Techniques
For more complex scenarios, advanced correlation techniques might be necessary:
-
Partial Correlation: This method helps assess the correlation between two variables while controlling for the influence of one or more other variables. It's useful for situations where confounding variables might obscure the true relationship.
-
Spearman's Rank Correlation: This non-parametric method is suitable for data that doesn't meet the assumptions of linear correlation, such as ordinal data or data with significant outliers. It measures the monotonic relationship between variables.
-
Kendall's Tau: Another non-parametric correlation measure, Kendall's tau is less sensitive to outliers than Spearman's rank correlation.
Practical Applications of Understanding Correlation
The ability to interpret correlation coefficients is crucial in numerous fields:
-
Finance: Analyzing the correlation between different assets to build diversified investment portfolios.
-
Healthcare: Studying the correlation between lifestyle factors and disease risk.
-
Social Sciences: Investigating the correlation between social factors and various outcomes.
-
Engineering: Assessing the correlation between different design parameters and performance metrics.
-
Climate Science: Examining the correlation between various climate variables and predicting future climate patterns.
Conclusion: The Importance of Context and Critical Thinking
Determining which r-value represents the strongest correlation is straightforward – the one with the highest absolute value. However, the interpretation of any correlation coefficient requires careful consideration of several factors beyond the numerical value itself. Context is crucial. Always visualize the data using scatter plots, consider potential confounding variables, assess the sample size, and acknowledge the limitations of the correlation coefficient. A critical and nuanced approach to interpreting correlation will lead to more accurate and insightful conclusions. By understanding the intricacies of correlation and employing appropriate statistical methods, you can unlock valuable insights from your data and make better-informed decisions. Remember that correlation does not equal causation – always maintain a healthy dose of skepticism and critical thinking when analyzing correlations.
Latest Posts
Latest Posts
-
How Long Should It Take To Read 50 Pages
May 12, 2025
-
What Is A Quadrilateral With One Pair Of Parallel Sides
May 12, 2025
-
A Clean Tie Attracts The Soup Of The Day
May 12, 2025
-
How Many Words Do You Say A Day
May 12, 2025
-
A Visible Saint Was The Term Puritans Used To Describe
May 12, 2025
Related Post
Thank you for visiting our website which covers about Which Of These R-values Represents The Strongest Correlation . We hope the information provided has been useful to you. Feel free to contact us if you have any questions or need further assistance. See you next time and don't miss to bookmark.