Which Value Of R Indicates A Stronger Correlation
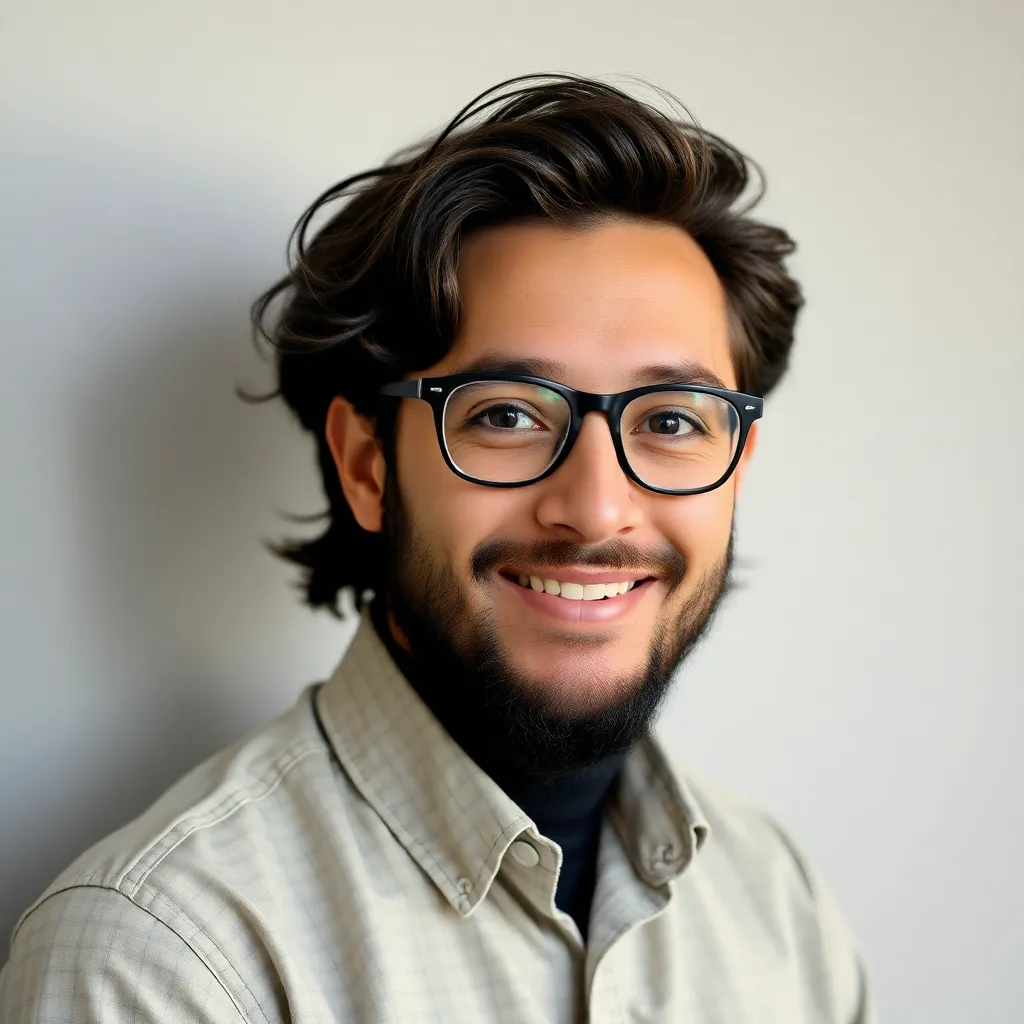
Arias News
May 10, 2025 · 6 min read
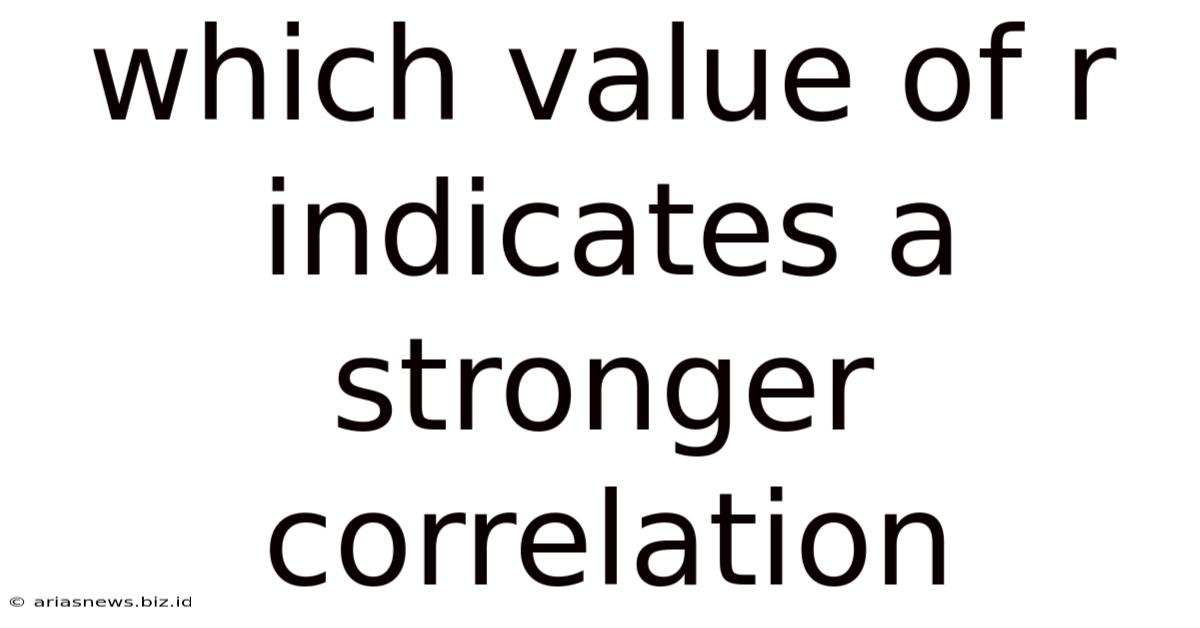
Table of Contents
Which Value of r Indicates a Stronger Correlation? Understanding Correlation Coefficients
Correlation is a fundamental concept in statistics that measures the strength and direction of a linear relationship between two variables. The correlation coefficient, often denoted by r, is a crucial statistic that quantifies this relationship. Understanding how different values of r indicate the strength of a correlation is essential for interpreting statistical analyses and drawing meaningful conclusions from data. This article will delve deep into the meaning of r, explaining how to interpret its values and highlighting the nuances involved.
Understanding the Correlation Coefficient (r)
The correlation coefficient r ranges from -1 to +1. This seemingly simple range holds a wealth of information about the relationship between two variables. Let's break down the different aspects:
The Magnitude of r: Strength of the Correlation
The absolute value of r reflects the strength of the linear relationship:
-
|r| close to 1: Indicates a strong linear correlation. The closer |r| is to 1, the stronger the relationship. This means that changes in one variable are closely associated with changes in the other variable in a predictable manner.
-
|r| around 0.7 to 0.9: Represents a moderately strong correlation. While not as precise as a strong correlation, a relationship still exists and is noticeable in the data.
-
|r| around 0.5 to 0.7: Indicates a moderate correlation. The relationship is noticeable but less predictable than moderately strong or strong correlations.
-
|r| around 0.3 to 0.5: Suggests a weak correlation. The relationship between variables is barely discernible amidst other factors influencing the data.
-
|r| close to 0: Indicates a very weak or no linear correlation. This doesn't necessarily mean there's no relationship at all; it simply suggests that there's no strong linear relationship. Other types of relationships, like non-linear ones, could still exist.
It's crucial to remember that correlation does not equal causation. Even a strong correlation doesn't prove that one variable causes changes in the other. There could be lurking variables, confounding factors, or pure coincidence at play.
The Sign of r: Direction of the Correlation
The sign of r indicates the direction of the linear relationship:
-
r > 0: Indicates a positive correlation. As one variable increases, the other tends to increase as well. This is often visualized as an upward-sloping line in a scatter plot. Examples include height and weight or study time and exam scores.
-
r < 0: Indicates a negative correlation. As one variable increases, the other tends to decrease. This is often visualized as a downward-sloping line in a scatter plot. Examples include exercise and body fat percentage or price of a product and quantity demanded.
-
r = 0: Suggests no linear correlation. The variables don't show a consistent upward or downward trend.
Interpreting r Values: Examples and Case Studies
Let's illustrate the interpretation of r with some examples:
Example 1: A study investigating the relationship between daily exercise and stress levels finds a correlation coefficient of r = -0.75. This indicates a moderately strong negative correlation. Increased daily exercise is associated with decreased stress levels.
Example 2: Research on ice cream sales and crime rates reveals a correlation coefficient of r = 0.82. This suggests a strong positive correlation. However, it's crucial to avoid the causal fallacy here. Higher ice cream sales don't cause higher crime rates. Both are likely influenced by a third variable, such as warmer weather.
Example 3: A study examining the relationship between shoe size and IQ scores finds a correlation coefficient of r = 0.10. This indicates a very weak correlation, suggesting that there's virtually no linear relationship between shoe size and intelligence.
Example 4: An analysis of the correlation between hours of sleep and academic performance yields r = 0.60. This represents a moderate positive correlation. More sleep is associated with better academic performance, although other factors contribute significantly.
Factors Influencing the Correlation Coefficient
Several factors can affect the correlation coefficient:
-
Outliers: Extreme data points can significantly distort the correlation coefficient. A single outlier can inflate or deflate the value of r, potentially misrepresenting the true relationship between the variables. Robust methods are often used to handle outliers, such as using Spearman's rank correlation instead of Pearson's correlation.
-
Non-linear relationships: The correlation coefficient only measures linear relationships. If the relationship between the variables is curved or non-linear, the value of r might be low even if a strong relationship exists. Scatter plots are essential for visualizing the relationship and identifying non-linear patterns.
-
Sample size: The reliability of the correlation coefficient increases with the sample size. Larger samples provide more accurate estimations of the population correlation. A small sample size can lead to unstable correlation estimates, making it challenging to draw robust conclusions.
-
Range restriction: If the range of values for one or both variables is restricted, the correlation coefficient might underestimate the true correlation. For example, if we only consider high-performing students, the correlation between study time and grades might appear weaker than if we included students with a wider range of academic performance.
Beyond Pearson's r: Other Correlation Measures
While Pearson's r is the most commonly used correlation coefficient, other measures exist to handle different types of data and relationships:
-
Spearman's rank correlation: This non-parametric method measures the monotonic relationship between two variables, regardless of whether the relationship is linear. It's particularly useful when dealing with ordinal data or when the assumption of normality is violated.
-
Kendall's tau: Another non-parametric correlation measure that assesses the ordinal association between two variables. It's often less sensitive to outliers than Spearman's rank correlation.
-
Point-biserial correlation: Used when one variable is continuous and the other is dichotomous (having only two categories).
The choice of correlation measure depends on the nature of the data and the research question.
Practical Applications and Importance of Understanding r
Understanding the correlation coefficient is crucial in various fields:
-
Finance: Analyzing the relationship between stock prices and market indices.
-
Healthcare: Investigating the correlation between lifestyle factors and disease risk.
-
Psychology: Studying the relationship between personality traits and behavior.
-
Education: Examining the correlation between teaching methods and student achievement.
-
Environmental Science: Analyzing the correlation between pollution levels and environmental health indicators.
Accurate interpretation of the correlation coefficient is vital for making informed decisions based on data analysis. Misinterpreting r can lead to flawed conclusions and inappropriate actions.
Conclusion: Interpreting r for Meaningful Insights
The correlation coefficient r is a powerful tool for quantifying the strength and direction of linear relationships between variables. However, its interpretation requires careful consideration of the magnitude and sign of r, potential confounding factors, and the limitations of the measure itself. By understanding the nuances of r and employing appropriate statistical methods, researchers and analysts can gain valuable insights from their data and make well-informed decisions. Always remember to visualize the data using scatter plots to gain a better understanding of the relationship beyond the numerical value of r. Furthermore, consider the context of your data and the limitations of correlation analysis when drawing conclusions. Don't fall into the trap of assuming causation simply because a strong correlation exists. The careful and nuanced interpretation of r is key to unlocking the true meaning hidden within your data.
Latest Posts
Latest Posts
-
The Square Root Of 27 Divided By 3 Is
May 11, 2025
-
Why Cant You Microwave Mcdonalds Coffee Cups
May 11, 2025
-
If I Was 40 What Year Was I Born
May 11, 2025
-
How To Say Little Brother In Spanish
May 11, 2025
-
How Many Gallons Are In A 15 Foot Round Pool
May 11, 2025
Related Post
Thank you for visiting our website which covers about Which Value Of R Indicates A Stronger Correlation . We hope the information provided has been useful to you. Feel free to contact us if you have any questions or need further assistance. See you next time and don't miss to bookmark.