A Correlation Coefficient Of 1.36 Would Be
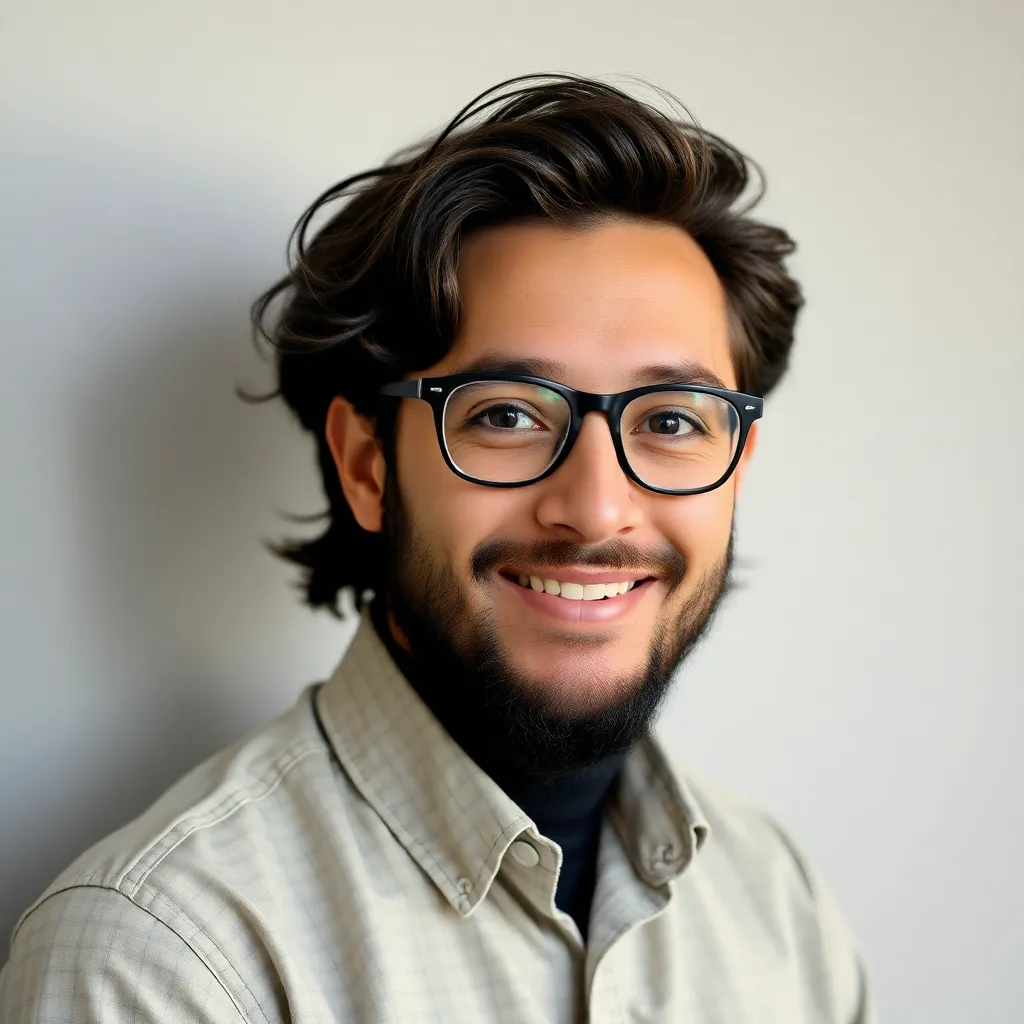
Arias News
May 11, 2025 · 6 min read
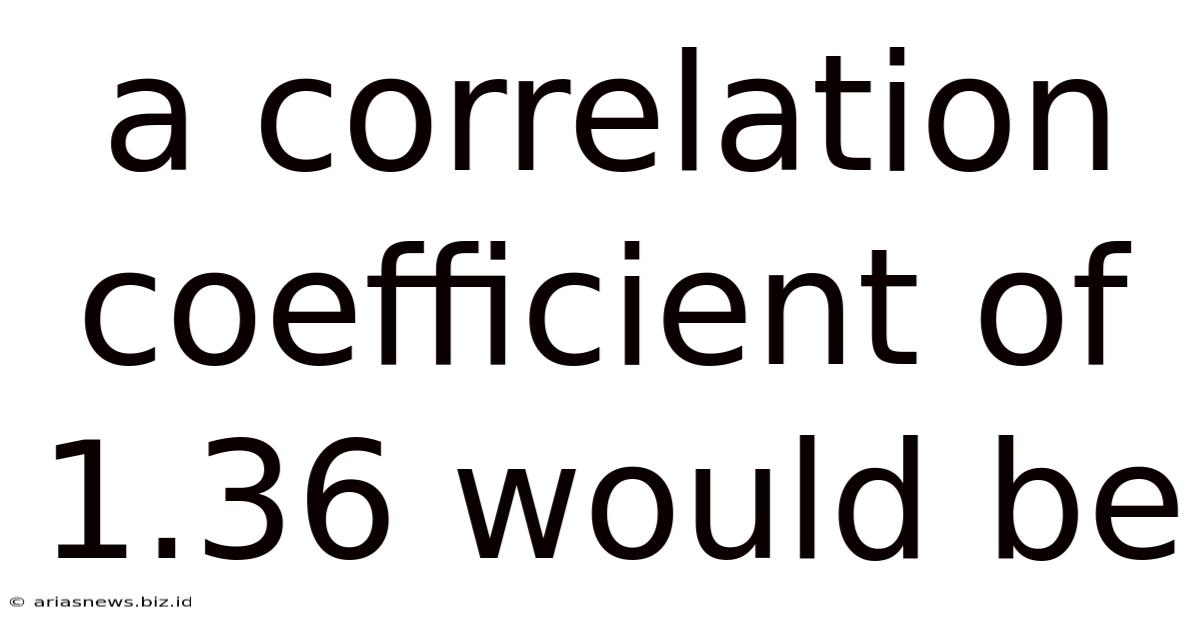
Table of Contents
A Correlation Coefficient of 1.36: Understanding the Impossible
A correlation coefficient of 1.36 is impossible. Correlation coefficients, typically represented by the letter r, measure the strength and direction of a linear relationship between two variables. They always fall within the range of -1 to +1. A value of +1 indicates a perfect positive correlation, -1 indicates a perfect negative correlation, and 0 indicates no linear correlation. Any value outside this range is simply not mathematically possible within the standard framework of correlation analysis.
This article will delve into why a correlation coefficient of 1.36 is erroneous, explore potential reasons for such an outcome, and discuss the importance of correctly interpreting correlation coefficients in research and data analysis. We'll also address how to handle such anomalies and ensure the accuracy of your statistical analyses.
Understanding Correlation Coefficients
Before examining the impossibility of a 1.36 correlation coefficient, let's solidify our understanding of what correlation coefficients represent. They provide a quantitative measure of the association between two variables. A positive correlation suggests that as one variable increases, the other tends to increase as well. Conversely, a negative correlation suggests that as one variable increases, the other tends to decrease. The absolute value of the coefficient indicates the strength of the relationship:
- |r| < 0.3: Weak correlation
- 0.3 ≤ |r| < 0.7: Moderate correlation
- |r| ≥ 0.7: Strong correlation
The sign (+ or -) denotes the direction of the relationship. A value of r = 0.8 indicates a strong positive correlation, while r = -0.8 indicates a strong negative correlation. Both represent strong relationships, but in opposite directions.
Why 1.36 is Impossible
The core issue with a correlation coefficient of 1.36 is that it exceeds the mathematically defined range of -1 to +1. This implies a fundamental error in the calculation or interpretation of the correlation. This error could stem from various sources, including:
-
Computational Errors: A simple mistake in the calculation process, perhaps a wrong formula used or an error in the input data, could lead to an impossible result. Double-checking the calculations using different software or methods is crucial.
-
Data Entry Errors: Inaccurate data entry is a common source of error in statistical analyses. Incorrect values or typos in the dataset can significantly distort the correlation coefficient. Careful data cleaning and validation are paramount.
-
Inappropriate Use of Correlation: Correlation analysis assumes a linear relationship between the variables. If the relationship is non-linear (e.g., curvilinear), a linear correlation coefficient will not accurately represent the association. Visualizing the data through scatter plots is essential to assess the relationship's nature.
-
Outliers: Extreme values (outliers) in the dataset can heavily influence the correlation coefficient, potentially leading to inflated or deflated values. Identifying and handling outliers appropriately is necessary for reliable results. Consider using robust correlation methods less sensitive to outliers.
-
Violation of Assumptions: Correlation analysis rests on certain assumptions, such as the variables following a bivariate normal distribution. Violation of these assumptions can lead to biased and inaccurate results. Assessing the normality of the data through histograms, Q-Q plots, and statistical tests is recommended.
Troubleshooting and Correcting Errors
Encountering a correlation coefficient like 1.36 necessitates a systematic investigation to identify and correct the underlying error. Here's a step-by-step approach:
-
Review the Data: Thoroughly examine the raw data for any errors, inconsistencies, or outliers. Check for typos, missing values, and unusual data points. Consider cleaning the data, imputing missing values, or transforming non-linear data.
-
Recalculate the Correlation: Recompute the correlation coefficient using a different statistical software package or method. This helps to identify potential errors in the initial calculations.
-
Visualize the Data: Create a scatter plot to visualize the relationship between the two variables. This will help to assess the linearity of the relationship and identify any outliers or unusual patterns.
-
Assess the Data Distribution: Check for normality in the data distributions using histograms and Q-Q plots. Consider applying data transformations (e.g., logarithmic or square root transformations) if the data is not normally distributed.
-
Consider Alternative Methods: If the relationship is non-linear, explore non-parametric correlation methods (e.g., Spearman's rank correlation) that don't assume linearity.
-
Consult Statistical Experts: If the problem persists, seeking assistance from a statistician or data analyst is advisable. They can provide valuable insights and guidance on handling complex data and statistical challenges.
Implications for Research and Data Analysis
The accurate calculation and interpretation of correlation coefficients are critical in various fields, including:
-
Social Sciences: Understanding correlations between social factors is essential for policy making and social intervention.
-
Medicine: Identifying correlations between risk factors and diseases is crucial for disease prevention and treatment.
-
Finance: Analyzing correlations between financial assets is crucial for risk management and investment decisions.
-
Engineering: Understanding correlations between design parameters and performance is vital for optimizing system design.
A flawed correlation coefficient can lead to inaccurate conclusions and inappropriate decisions. Therefore, meticulous attention to detail and careful data analysis are crucial for ensuring the validity and reliability of research findings.
Beyond Linear Correlation: Exploring Other Relationships
While a correlation coefficient of 1.36 points to an error in a linear correlation analysis, the variables might still exhibit a strong relationship, but not a linear one. It's essential to explore alternative analytical approaches to understand the association between variables fully. This might include:
-
Non-linear Regression: If the scatter plot reveals a curvilinear relationship, non-linear regression models can be used to model the association. These models can capture complex relationships that linear models cannot.
-
Spearman's Rank Correlation: This non-parametric method measures the monotonic relationship between two variables, meaning it assesses whether the variables tend to move in the same direction, even if the relationship isn't strictly linear.
-
Kendall's Tau Correlation: Similar to Spearman's correlation, Kendall's tau also measures monotonic relationships but is less sensitive to outliers.
-
Partial Correlation: This technique assesses the correlation between two variables while controlling for the effect of one or more other variables. This is useful when confounding variables might influence the observed relationship.
The Importance of Data Visualization
Visualizing your data, especially through scatter plots, is a crucial step in any correlation analysis. A scatter plot can reveal patterns and anomalies that might not be apparent from just the correlation coefficient. It can immediately highlight:
-
Non-linear relationships: Curves or other non-linear patterns in the scatter plot indicate that a linear correlation coefficient is inappropriate.
-
Outliers: Points far removed from the main cluster of data points are easily identified and can be investigated for potential errors or unusual circumstances.
-
Clusters: The data may show distinct clusters, suggesting a more complex relationship than a simple linear correlation can capture.
Conclusion: Ensuring Accuracy in Correlation Analysis
A correlation coefficient of 1.36 is mathematically impossible within the standard framework of correlation analysis. This highlights the importance of meticulous data handling, careful calculation, and appropriate interpretation of statistical results. By systematically investigating potential errors, visualizing the data, and considering alternative analytical approaches, researchers and analysts can ensure the accuracy and reliability of their correlation analyses, leading to valid and insightful conclusions. Remember that statistical analysis is a tool, and its effective use requires a deep understanding of its limitations and assumptions. Always prioritize data integrity and critical evaluation of results to avoid misinterpretations and flawed conclusions. Using a combination of statistical techniques and careful data visualization is key to uncovering meaningful relationships within your data.
Latest Posts
Latest Posts
-
18 Is 30 Percent Of What Number
May 12, 2025
-
How Do You Say Chicken Butt In Spanish
May 12, 2025
-
How Long Should It Take To Read 50 Pages
May 12, 2025
-
What Is A Quadrilateral With One Pair Of Parallel Sides
May 12, 2025
-
A Clean Tie Attracts The Soup Of The Day
May 12, 2025
Related Post
Thank you for visiting our website which covers about A Correlation Coefficient Of 1.36 Would Be . We hope the information provided has been useful to you. Feel free to contact us if you have any questions or need further assistance. See you next time and don't miss to bookmark.