The Information Collected During An Experiment Is Called
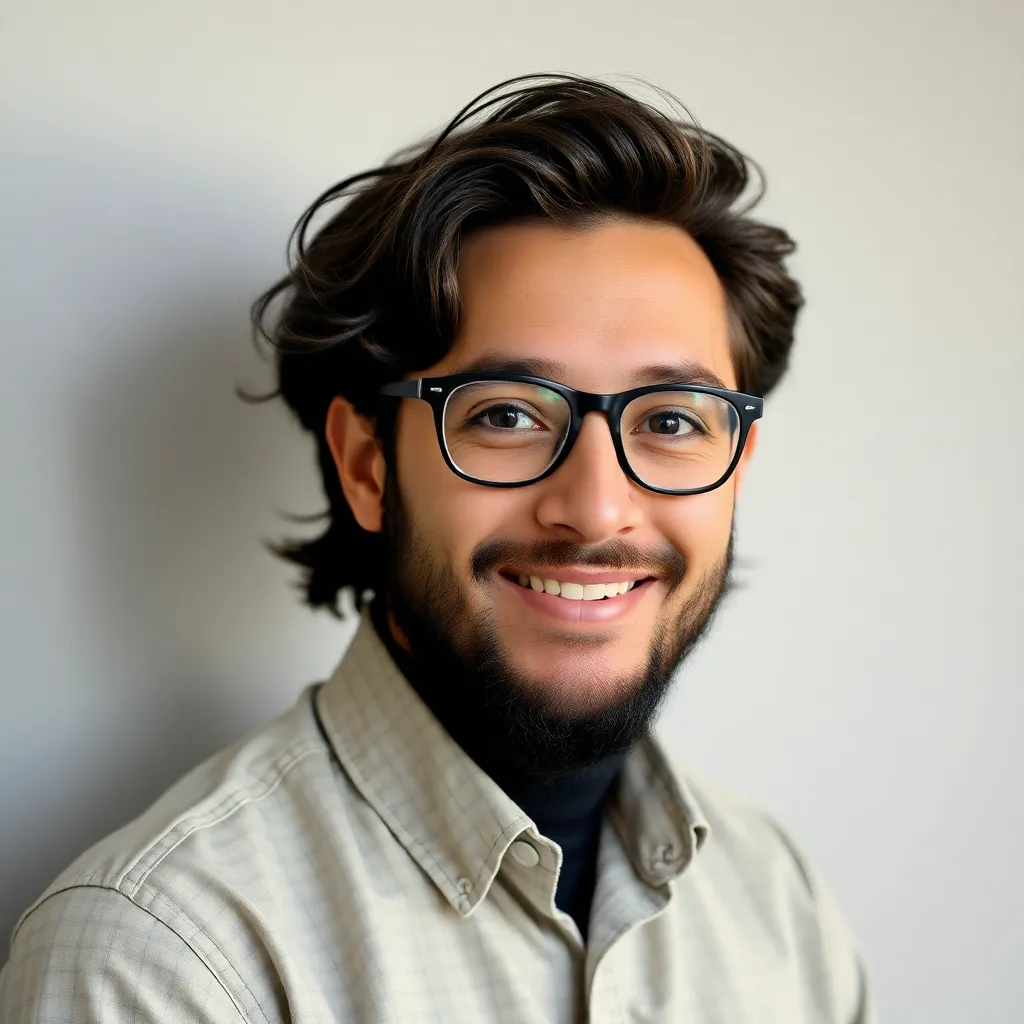
Arias News
May 11, 2025 · 6 min read
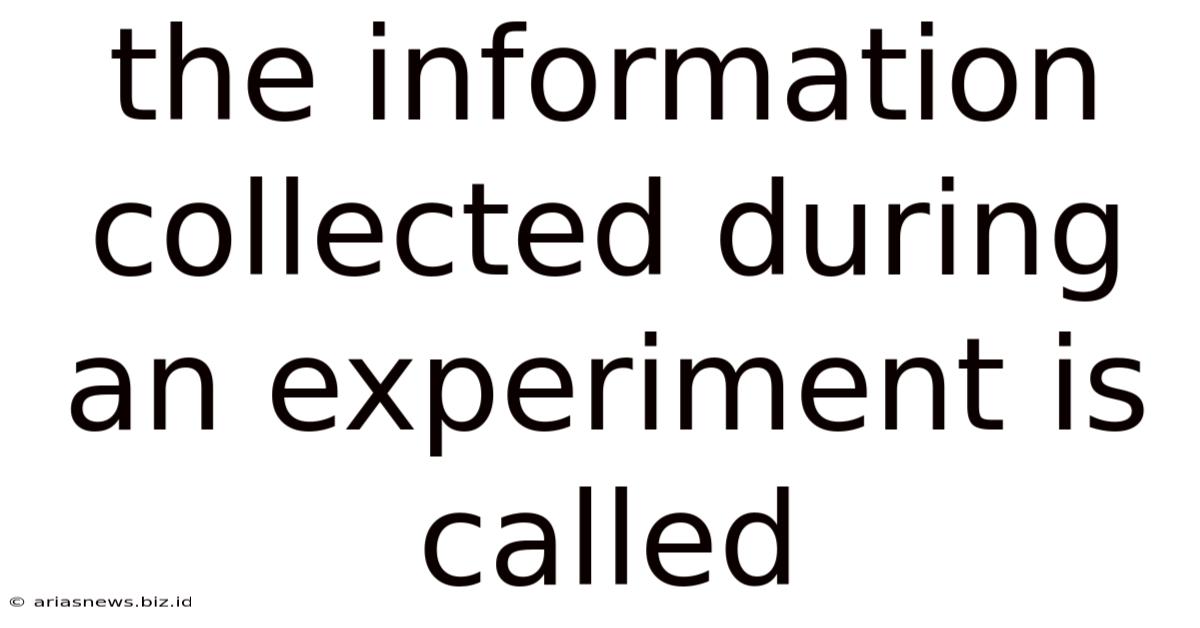
Table of Contents
The Information Collected During an Experiment is Called: Data – A Deep Dive into Experimental Design and Analysis
The bedrock of any scientific endeavor, be it in physics, biology, psychology, or sociology, lies in the meticulous collection and analysis of data. But what exactly is this data, and how does its nature influence the entire experimental process? This comprehensive guide will explore the different types of data collected during experiments, their importance in drawing conclusions, and the crucial role they play in scientific validity and reproducibility.
Understanding "Data" in the Context of Experiments
The information collected during an experiment is called data. This is a broad term encompassing any and all observations, measurements, and recordings made during the experimental process. It’s the raw material from which scientific knowledge is built. Data can take many forms, and understanding these different forms is critical to proper experimental design and analysis.
The Importance of Accurate and Reliable Data Collection
The quality of your experiment hinges entirely on the accuracy and reliability of your data. Inaccurate or unreliable data can lead to flawed conclusions, wasted resources, and even the propagation of misinformation. Therefore, meticulous data collection methods, including:
- Precise Measurement Techniques: Utilizing calibrated instruments and employing standardized procedures.
- Careful Observation and Recording: Maintaining detailed records of observations, including any anomalies or unexpected events.
- Data Validation and Verification: Implementing checks and balances to ensure data accuracy and consistency.
are essential for a successful experiment.
Types of Data Collected During Experiments
Data can be broadly categorized into two main types:
1. Qualitative Data
Qualitative data describes qualities or characteristics. It's non-numerical and often involves observations, descriptions, and interpretations. Examples include:
- Observations of Behavior: Recording the behaviors of animals in their natural habitat.
- Interviews and Focus Groups: Gathering subjective opinions and experiences from participants.
- Textual Analysis: Analyzing documents, transcripts, or other textual data to identify themes and patterns.
- Case Studies: Detailed investigations of individual cases or events.
Qualitative data is crucial for exploring complex phenomena and gaining a deep understanding of context. While it may not be directly quantifiable, it often provides valuable insights that inform quantitative research. Analyzing qualitative data frequently involves techniques like thematic analysis, grounded theory, and content analysis.
2. Quantitative Data
Quantitative data is numerical and can be measured and analyzed statistically. This type of data is often collected using instruments or standardized scales. Examples include:
- Measurements of Physical Quantities: Recording temperature, weight, length, or other physical properties.
- Survey Responses: Gathering numerical data through questionnaires with scaled responses (e.g., Likert scales).
- Experimental Outcomes: Measuring the effects of a treatment or intervention on a dependent variable.
- Counts and Frequencies: Recording the number of times an event occurs.
Quantitative data allows for statistical analysis, enabling researchers to identify trends, correlations, and causal relationships. Statistical methods, such as t-tests, ANOVA, regression analysis, and correlation analysis, are commonly used to analyze this data.
The Relationship Between Data Types and Experimental Design
The type of data you collect will significantly influence the experimental design you choose. For instance:
- Experimental Designs for Quantitative Data: Experiments designed to collect quantitative data often involve controlled environments, precise measurements, and large sample sizes to ensure statistical power. Examples include randomized controlled trials (RCTs) and factorial designs.
- Experimental Designs for Qualitative Data: Experiments focusing on qualitative data may employ methods such as ethnographic studies, case studies, or participatory action research, emphasizing in-depth understanding and context.
Choosing the appropriate experimental design ensures that the collected data is relevant, reliable, and suitable for the chosen analytical methods.
Data Collection Methods
The methods used to collect data vary depending on the type of data and the research question. Some common methods include:
- Surveys: Questionnaires administered to a sample population to gather information on attitudes, opinions, and behaviors.
- Experiments: Controlled studies designed to investigate the relationship between variables.
- Observations: Systematic recording of behaviors or events in a natural or controlled setting.
- Interviews: Direct conversations with individuals to gather in-depth information.
- Document Analysis: Examination of existing documents (e.g., records, reports, articles) to extract relevant information.
- Focus Groups: Group discussions used to explore a particular topic or issue.
- Sensors and Instruments: Using technological devices to collect objective data (e.g., temperature sensors, accelerometers).
Data Cleaning and Preprocessing
Before analyzing data, it's crucial to clean and preprocess it. This involves several steps:
- Identifying and Handling Missing Data: Dealing with incomplete or missing data points, using methods such as imputation or exclusion.
- Dealing with Outliers: Identifying and managing extreme data points that may skew the results.
- Data Transformation: Converting data into a suitable format for analysis, such as standardizing or normalizing variables.
- Data Validation: Checking for errors and inconsistencies in the data.
Proper data cleaning ensures that the subsequent analysis yields accurate and reliable results.
Data Analysis and Interpretation
Once the data is cleaned and preprocessed, it's ready for analysis. The choice of analytical techniques depends on the type of data and the research question. Some common methods include:
- Descriptive Statistics: Summarizing data using measures like mean, median, mode, standard deviation, and frequency distributions.
- Inferential Statistics: Making inferences about a population based on a sample of data. This includes hypothesis testing and confidence intervals.
- Regression Analysis: Examining the relationship between a dependent variable and one or more independent variables.
- Correlation Analysis: Assessing the strength and direction of the relationship between two variables.
- Qualitative Data Analysis: Analyzing themes, patterns, and meanings in qualitative data through methods like coding, thematic analysis, and grounded theory.
Reporting and Communicating Results
The final step in the process is reporting and communicating the results of the analysis. This involves clearly presenting the data, the methods used, and the conclusions drawn. Effective communication of research findings is crucial for disseminating knowledge and influencing practice. Key elements of a good research report include:
- Clear and Concise Writing: Using simple and unambiguous language to convey information effectively.
- Visual Aids: Utilizing graphs, charts, and tables to illustrate key findings.
- Transparent Methodology: Clearly describing the methods used for data collection and analysis.
- Discussion of Limitations: Acknowledging limitations of the study and potential sources of bias.
- Implications and Recommendations: Discussing the implications of the findings and offering recommendations for future research or practice.
The Role of Data in Scientific Rigor and Reproducibility
The accurate and reliable collection of data is paramount for maintaining scientific rigor and reproducibility. Detailed documentation of the data collection process, including methods, instruments used, and any relevant contextual information, allows others to replicate the study and verify the findings. This transparency is crucial for building trust in scientific research and advancing scientific knowledge.
Conclusion: Data – The Foundation of Scientific Discovery
In conclusion, the information collected during an experiment – the data – is the lifeblood of scientific inquiry. Understanding the different types of data, employing appropriate collection methods, performing rigorous analysis, and transparently communicating results are all essential for conducting robust and impactful research. From the precise measurements of physical quantities to the nuanced interpretations of qualitative observations, data forms the foundation upon which scientific discoveries are made and our understanding of the world is continually refined. The meticulous attention to data, from its collection to its interpretation, underpins the reliability and validity of any scientific finding, contributing to the advancement of knowledge across various disciplines.
Latest Posts
Latest Posts
-
95 Out Of 100 As A Percentage
May 12, 2025
-
Is A Monitor An Output Or Input
May 12, 2025
-
What Are All The Factors Of 11
May 12, 2025
-
Which Polygon Has An Interior Angle Sum Of 1080
May 12, 2025
-
Difference Between 100 Meters And 100 Yards
May 12, 2025
Related Post
Thank you for visiting our website which covers about The Information Collected During An Experiment Is Called . We hope the information provided has been useful to you. Feel free to contact us if you have any questions or need further assistance. See you next time and don't miss to bookmark.