Which Option Best Completes The Table 6.3.2
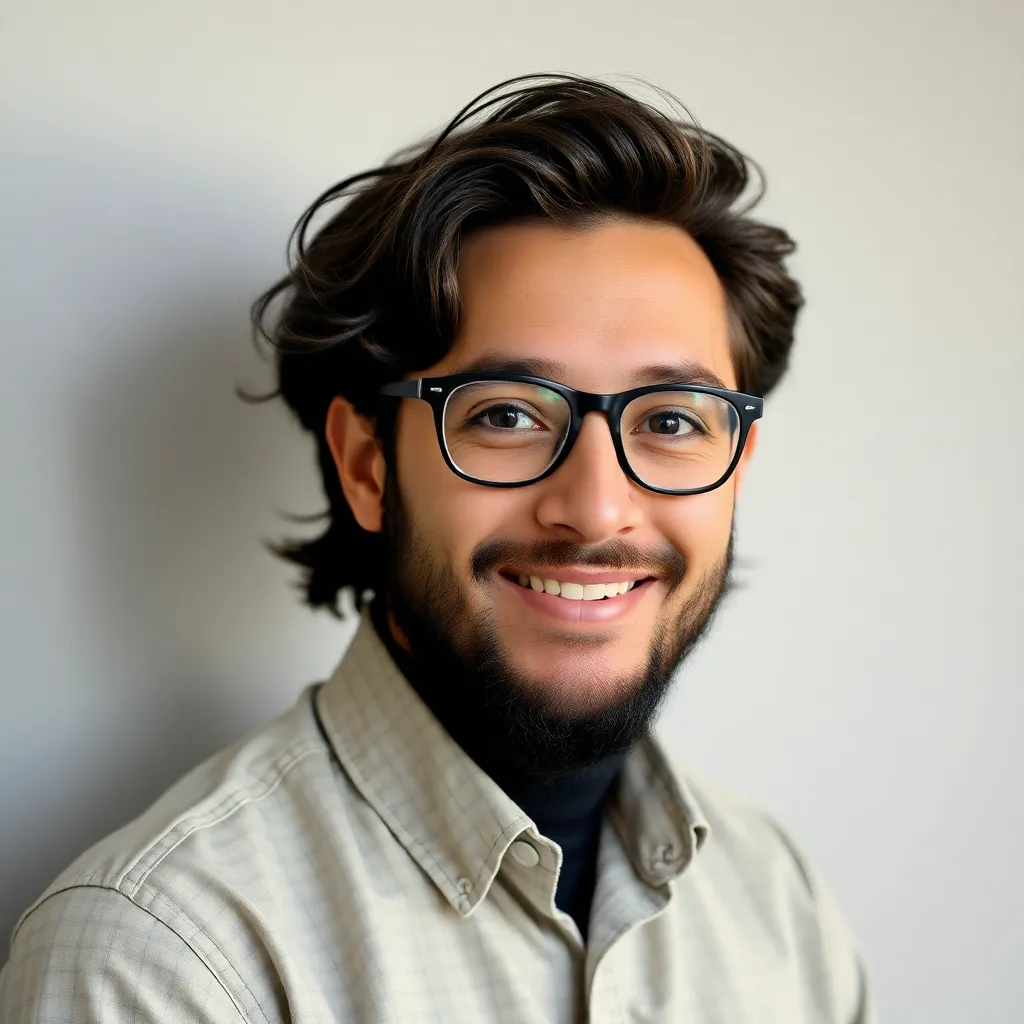
Arias News
Apr 01, 2025 · 5 min read
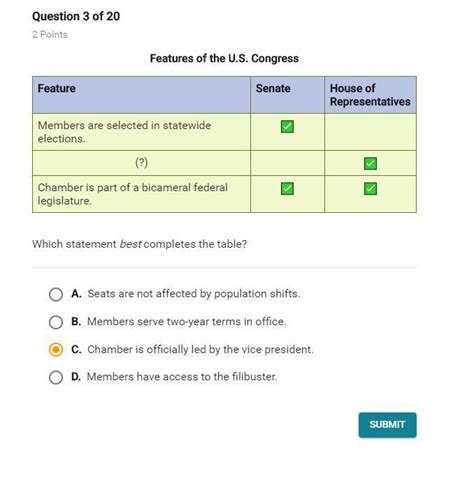
Table of Contents
Which Option Best Completes Table 6.3.2? A Comprehensive Guide to Data Analysis and Table Completion
This article delves into the crucial task of completing tables, specifically focusing on the question, "Which option best completes Table 6.3.2?" This seemingly simple question underpins a broader range of complex data analysis techniques and critical thinking skills. We'll explore various approaches to determine the optimal solution, considering the context, data patterns, and logical reasoning necessary to arrive at the most accurate and informed answer.
While we don't have the actual Table 6.3.2 to analyze directly (as it's not provided), we'll explore several hypothetical scenarios and methodologies applicable to a wide range of table completion problems. This practical approach will enable you to apply these techniques to your specific table.
Understanding the Context: The Foundation of Table Completion
Before diving into methods for completing Table 6.3.2, understanding the context is paramount. Ask yourself these vital questions:
-
What is the table's purpose? Is it summarizing experimental results, presenting financial data, demonstrating population statistics, or something else entirely? The table's objective significantly influences how we approach filling in missing values.
-
What are the variables involved? Identify the independent and dependent variables (if applicable). Understanding the relationship between variables helps in predicting missing data points.
-
What is the nature of the data? Is it numerical (continuous or discrete), categorical, or a combination of both? The data type dictates the appropriate analytical methods.
-
What are the units of measurement? Consistent units are crucial for accurate analysis and interpretation.
-
Are there any known relationships or patterns within the data? Recognizing trends, correlations, or sequences can guide the completion process.
Methodologies for Completing Tables: From Simple to Sophisticated
The method used to complete Table 6.3.2 depends heavily on its content and the nature of the missing data. Let’s examine several common approaches:
1. Simple Arithmetic and Pattern Recognition:
This approach is useful when the table reveals clear arithmetic sequences or patterns. For instance, if a column shows a consistent increase or decrease, simple addition or subtraction can be used to predict the missing value. Similarly, if the table represents a geometric progression, we can identify the common ratio and extrapolate to find the missing entry.
Example: If a column shows values 2, 4, 6, __, 10, the missing value is clearly 8, following a simple arithmetic progression.
2. Averages and Statistical Measures:
When dealing with numerical data, calculating averages (mean, median, mode) can help estimate missing values. The choice of average depends on the data distribution. For instance, the median is less sensitive to outliers than the mean.
Example: If a row contains values 10, 15, __, 25, and the average is known to be 18, we can infer the missing value.
3. Linear Regression and Interpolation:
If the relationship between variables is linear (or can be reasonably approximated as such), linear regression can be used to predict missing values. This method involves fitting a straight line to the existing data points and using the equation of the line to predict values for missing data points. Interpolation, a similar technique, can be used to estimate values within the range of existing data. Note that extrapolation (predicting beyond the existing range) is generally less reliable.
Example: If a table plots temperature versus time, and we have data for several time points, linear regression can be used to predict the temperature at an intermediate time point.
4. Data Imputation Techniques:
For more complex scenarios with missing data, advanced statistical imputation techniques can be employed. These methods aim to replace missing values with plausible estimates based on the available data. Common imputation techniques include:
-
Mean imputation: Replacing missing values with the mean of the observed values. This is a simple but potentially biased method.
-
Median imputation: Replacing missing values with the median of the observed values. More robust to outliers than mean imputation.
-
Mode imputation: Replacing missing values with the most frequent value. Suitable for categorical data.
-
Regression imputation: Using regression models to predict missing values based on other variables. A more sophisticated approach.
-
Multiple Imputation: Creating multiple plausible imputed datasets and analyzing them separately, providing a more robust estimate considering the uncertainty introduced by missing values.
5. Logical Reasoning and Contextual Information:
Sometimes, the best way to complete a table involves applying logical reasoning and considering the contextual information provided. This might involve interpreting relationships between different variables, identifying patterns not readily apparent through mathematical calculations, or using external knowledge to fill in the gaps.
Example: If the table concerns the population of different cities, and one value is missing, considering geographical proximity and population density of neighboring cities might help to estimate the missing population.
Analyzing the "Best" Option: Considerations and Criteria
Once you've used one or more of the above methods, you need to assess which option is "best." This involves considering various factors:
-
Accuracy: How close is the estimated value to the true value (if known)? Consider potential error margins.
-
Consistency: Does the completed table maintain internal consistency? Are the relationships between variables plausible and consistent with the overall pattern?
-
Plausibility: Does the completed table make sense in the context of the problem? Are the values realistic and reasonable?
-
Methodological Justification: Can you clearly justify the method used to complete the table? Explain your reasoning and assumptions clearly.
-
Sensitivity Analysis: Explore how sensitive the completed table is to changes in the assumptions or the method used. If small changes lead to large variations in the result, this may indicate a less reliable completion.
Conclusion: A Multifaceted Approach to Table Completion
Determining which option best completes Table 6.3.2 is not a simple task. It requires a multifaceted approach that integrates various analytical techniques, a strong understanding of the data's context, and careful consideration of the criteria for evaluating different options. By employing the methods outlined in this article, from simple arithmetic to advanced imputation techniques, and carefully weighing the factors discussed above, you can confidently and effectively complete the table and draw meaningful conclusions from the data. Remember that transparency and justification of your approach are crucial for validating your findings and ensuring the reliability of your analysis. The process often requires iterative refinement and may involve revisiting your methodology based on your insights as you proceed.
Latest Posts
Latest Posts
-
What Does 925 Sun Mean On Jewelry
Apr 02, 2025
-
How Many Fingers Does A Turtle Have
Apr 02, 2025
-
How Many Ounces In A Slice Of Bread
Apr 02, 2025
-
What Percentage Of An Hour Is 45 Minutes
Apr 02, 2025
-
What Were Mattresses Made Of In The 1300s
Apr 02, 2025
Related Post
Thank you for visiting our website which covers about Which Option Best Completes The Table 6.3.2 . We hope the information provided has been useful to you. Feel free to contact us if you have any questions or need further assistance. See you next time and don't miss to bookmark.